Artificial Intelligence: How to get it right
Published 28 January 2022
Putting policy into practice for safe data-driven innovation in health and care
Joshi, I., Morley, J.,(eds) (2019). London, United Kingdom: NHSX.
Ministerial foreword
We love the NHS because it’s always been there for us, through some of the best moments in life and some of the worst. That’s why we’re so excited about the extraordinary potential of artificially intelligent systems (AIS) for healthcare.
Put simply, this technology can make the NHS even better at what it does: treating and caring for people.
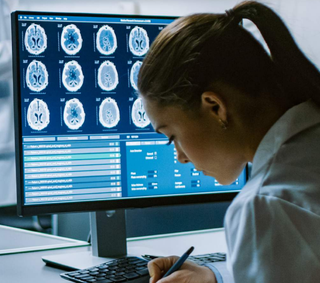
This includes areas like diagnostics, using data-driven tools to complement the expert judgement of frontline staff. In the report, for example, you’ll read about the East Midlands Radiology Consortium who are studying Artificial Intelligence (AI) as a ‘second reader’ of mammogram images, helping radiologists with an incredibly consequential decision, whether or not to recall a patient. In the near future this kind of tech could mean faster diagnosis, more accurate treatments, and ultimately more NHS patients hearing the words ‘all clear’.
AI can also help us get smarter in the way we plan the NHS and manage its resources. Take NHS Blood & Transplant, who are looking at how AI can forecast how much blood plasma a hospital needs to hold onsite on any given day. Or University College London Hospitals (UCLH) who are trialling tools that can predict the risk of missed outpatient appointments.
Most exciting of all is the possibility that AI can help with the next round of game-changing medical breakthroughs. Already, algorithms can compare tens of thousands of drug compounds in a matter of weeks instead of the years it would take a human researcher. Genomic data could radically improve our understanding of disease and help us get better at taking pre-emptive action that keeps people out of hospitals.
But while the opportunities of AI are immense so too are the challenges.
Much of the NHS is locked into ageing technology that struggles to install the latest update, never mind the latest AI tools, so we need a strong focus on fixing the basic infrastructure. That means sorting out the connectivity, standardising the data and replacing our siloed and fragmented systems with systems that can talk to each other. We also need to make sure that staff have the skills, training and support to feel confident in using or procuring emerging technology.
Just as important, as a society we need to agree the rules of the game. If we want people to trust this tech, then ethics, transparency and the founding values of the NHS have to got to run through our AI policy like letters through a stick of rock.
And while we’re clear-eyed about the promise of AI we can’t let ourselves be blinded by the hype (of which this field has more than its fair share). Our focus has got to be on demonstrably effective tech that can make a practical difference, at scale, right across the NHS, not just the country’s most advanced teaching hospitals.
To help us deliver those changes, we’ve set up NHSX, a new joint team working across the NHS family to accelerate the digitisation of health and care. NHSX’s job is to build the ecosystem in which healthtech innovation can flourish for the benefit of the NHS. Crucially it’s also been tasked with doing this in the right way, within a standardised, ethically and socially acceptable framework.
Getting these foundations right matters hugely, which is why we are investing £250 million in the creation of the NHS AI Lab to focus on supporting innovation in an open environment where innovators, academics, clinicians and others can develop, learn, collaborate and build technologies at scale to deliver maximum impact in health and care safely and effectively.
The NHS AI Lab will be run collaboratively by NHSX and the Accelerated Access Collaborative and will encompass work programmes designed to:
- Accelerate adoption of proven AI technologies e.g. image recognition technologies including mammograms, brain scans, eye scans and heart monitoring for cancer screening.
- Encourage the development of AI technologies for operational efficiency purposes e.g. predictive models that better estimate future needs of beds, drugs, devices or surgeries.
- Create environments to test the safety and efficacy of technologies that can be used to identify patients most at risk of diseases such as heart disease or dementia, allowing for earlier diagnosis and cheaper, more focused, personalised prevention.
- Train the NHS workforce of the future so that they can use AI systems for day-to-day tasks.
- Inspect algorithms already used by the NHS, and those being developed for the NHS, to increase the standards of AI safety, making systems fairer, more robust and ensuring patient confidentiality is protected.
- Invest in world-leading research tools and methods that help people apply ethics and regulatory requirements.
The following report sets out the foundational policy work that has been done in developing the plans for the NHS AI Lab. It also shows why we’re so hopeful about the future of the NHS.
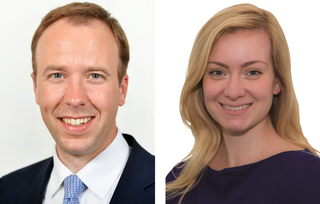
Executive summary
The potential of AI
Artificial Intelligence could help personalise NHS screening and treatments for cancer, eye disease and a range of other conditions, for example, while freeing up staff time to spend with patients.
Artificial Intelligence (AI) has the potential to make a significant difference to health and care. A broad range of techniques can be used to create Artificially Intelligent Systems (AIS) to carry out or augment health and care tasks that have until now been completed by humans, or have not been possible previously; these techniques include inductive logic programming, robotic process automation, natural language processing, computer vision, neural networks and distributed artificial intelligence.
These technologies present significant opportunities for keeping people healthy, improving care, saving lives and saving money for the pilot digital technologies. It could help personalised NHS screening and treatments for cancer, eye disease and a range of other conditions, for example. Furthermore, it’s not just patients who can benefit. AI can also support clinicians, enabling them to make the best use of their expertise, informing their decisions and saving them time.
This report gives a considered and cohesive overview of the current state of play of data-driven technologies within the health and care system, covering everything from the local research environment to international frameworks in development. Informed by research conducted by NHSX and other partners over the past year, it outlines where in the system AI technologies can be utilised and the policy work that is, and will need to be done, to ensure this utilisation is done in a safe, effective and ethically acceptable manner. Specifically:
Chapters 1 and 2 set the scene
They provide an overview of what AI is (and importantly is not), why we believe it is important, and a detailed look at what is currently being developed by the AI ecosystem by evaluating the results of a horizon scanning exercise and our second ‘State of the Nation’ survey. This analysis reveals that diagnosis and screening are the most common uses of AI, with 132 different AI products identified being designed for diagnosis or screening purposes covering 70 different conditions.
Chapter 3 is an in-depth look at the Governance of AI
Building on the Code of Conduct for data-driven technologies, it explores the development of a novel governance framework that emphasises both the softer ethical considerations of the “should vs should not” in the development of AI solutions as well as the more legislative regulations of “could vs could not”. In particular it covers key areas such as the explainability of an algorithm, the evidence generation for efficacy of fixed algorithms, the importance of patient safety and what to consider in commercial strategies.
Chapter 4 is all about the data that fuels AI
When engaging with innovators, regulators, commissioners and citizens on AI the one topic that is guaranteed to come up is Information Governance (IG). Protecting patient data is of the utmost importance, which is why IG is crucial, but it should not be seen as a blocker to the use of data for purposes that can deliver genuine benefits to patients, clinicians and the system. This Chapter highlights how we are working collaboratively with key partners across the system (e.g. the Accelerated Access Collaborative, the Office of Life Sciences, Health Data Research UK, Genomics England, Academic Health Science Network) to clarify the rules of IG and streamline access to data for good through specific programmes such as the Digital Innovation Hubs.
Chapter 5 covers adoption and spread
Considering the sometimes negative impact the complexity of the NHS as a sociotechnical system has on the spread of important innovation, it covers the actions being taken to encourage adoption. However, given the challenges involved in the practical implementation of AI we do not want to encourage adoption for the sake of adoption, so it also covers ‘what good looks like’ and how we can monitor the impact of the introduction of AI over time so that good stays good further downstream.
Chapter 6 comes back to the people of the NHS
Building on the work of Health Education England and the Topol Review, it highlights the challenges faced by the workforce in the development, deployment and use of AI and what needs to be done in order to ensure they have the skills that they need to feel confident in using AI in clinical practice safely and effectively. Crucially, it highlights how again we cannot do this alone and must work closely with national centres of data science training such as the Alan Turing Institute.
Chapter 7 goes international
Health data is not only generated in England and the AI technologies that are trained and tested on it are not developed only in England. Instead the AI ecosystem is truly international and there is, therefore, a need for international collaboration and agreement of standards, frameworks and guidance. For this reason, this chapter highlights the ongoing work of the Global Digital Health Partnership, the World Health Organisation and the EQUATOR network in developing these with us as a key partner.
Chapter 8 concludes with the NHS AI Lab
It brings together all the information included in the previous chapters to highlight why we know that the Lab is needed and why we think it will be crucial in helping us achieve our aims of:
- promoting the UK as the best place in the world to invest in healthtech
- providing evidence of what good practice looks like to industry and commissioners
- reassuring the public, patients and clinicians that data-driven technology is safe, effective and protects privacy
- allowing the government to work with suppliers to guide the development of new technology so products are suitable for the health and care system in the future
- building capability within the system with In-house expertise to prototype and develop ideas
- making sure the NHS gets a fair deal from the commercialisation of its data resources and expertise.
Introduction
(Dr Indra Joshi and Jessica Morley)
Definition
Despite being a well-established field of computer science research, Artificial Intelligence (AI) is difficult to define and, as such, numerous definitions exist, including:
- “the designing and building of intelligent agents that receive precepts from the environment and take actions that affect that environment”1
- “a cross-disciplinary approach to understanding, modelling, and replicating intelligence and cognitive processes invoking various computational, mathematical, logical, mechanical, and even biological principles and devices”2
- “the science of making machines do things that would require intelligence if done by people”3
The third definition is the oldest, stemming from the field’s founding document “Proposal for the Dartmouth Summer Research Project on Artificial Intelligence” (1955). However, it is the most applicable to the uses of Artificial Intelligence for health and social care.
Opportunities
In the context of health and care, a broad range of techniques (e.g. inductive logic programming, robotic process automation, natural language processing, computer vision, neural networks and distributed artificial intelligence such as agent based modelling4) are used to create Artificially Intelligent Systems (AIS) that can carry out medical tasks traditionally done by professional healthcare practitioners. The number of medical or care-related tasks that can be automated or augmented in this manner is significant.
A summary of the areas of care in which such automated tasks could make a difference is presented below.
-
Diagnostics
Image recognition
Symptoms checkers and decision support
Risk stratification
-
Knowledge generation
Drug discovery
Pattern recognition
Greater knowledge of rare diseases
Greater understanding of casuality
-
Public health
Digital epidemiology
National screening programmes
-
System efficiency
Optimisation of care pathways
Prediction of Do Not Attends
Identification of staffing requirements
-
P4 medicine
Prediction of deterioration
Personalised treatments
Preventative advice
This range of potential use cases for AI in health and care highlights the scale of the opportunity presented by AI for the health and care sector.
This is why:
- The NHS Long-Term Plan sets out the ambition to use decision support and AI to help clinicians in applying best practice, eliminate unwarranted variation across the whole pathway of care, and support patients in managing their health and condition.
- The future of healthcare: our vision for digital, data and technology in health and care outlines the intention to use cutting-edge technologies (including AI) to support preventative, predictive and personalised care.
- The Industrial Strategy AI Mission sets the UK the target of “using data, Artificial Intelligence and innovation to transform the prevention, early diagnosis and treatment of chronic diseases by 2030.”
We believe that the UK can be a world leader in this area for years to come - a core aim of the Office for Artificial Intelligence (OAI).
Ethics and safety
There are significant ethical and safety concerns associated with the use of AI in health and care.
Challenges
As much as we believe in the power of AI to deliver significant benefits to health and care, and the wider economy, we also know that there are significant ethical and safety concerns associated with the use of AI in health and care.
If we do not think about transparency, accountability, liability, explicability, fairness, justice and bias, it is possible that increasing the use of data-driven technologies, including AI, within the health and care system could cause unintended harm.
Tackling these challenges so that the opportunities can be capitalised on, and the risks mitigated, requires taking action in five key areas:
- Leadership & Society: creating a strong dialogue between industry, academia, and Government.
- Skills & Talent: developing the right skills that will be needed for jobs of the future and that will contribute to building the best environment for AI development and deployment.
- Access to Data: facilitating legal, fair, ethical and safe data sharing that is scalable and portable to stimulate AI technology innovation.
- Supporting Adoption: driving public and private sector adoption of AI technologies that are good for society.
- International engagement: securing partnerships that deliver access to scale for our ecosystem.
This report sets out current and future developments in each of these areas, and provides the rationale for why NHSX is creating the new £250 million NHS AI Lab in collaboration with the Accelerated Access Collaborative (AAC). Overall the goal is to help the system players from innovators to commissioners, to fully harness the benefits of AI technologies within safe and ethical boundaries, whilst speeding up the development, deployment and use of AI so that we can get benefits to more people - patients and staff alike - more quickly.
Where are we now?
(Jessica Morley, Marie-Anne Demestihas, Sile Hertz, Ian Newington & Mike Trenell.)
As a starting point, we needed to understand the baseline that we were working from. In order to develop useful frameworks and focus investment, we needed to understand what is:
a) The current state of AI in the health and care system i.e. hype vs reality;
b) The challenges faced by innovators in developing AI systems;
c) The issues faced by policy makers and regulators in governing both the development and deployment of AI systems in health.
Two activities were carried out to get an up-to-date picture of AI solutions that are available on the market and where support is needed to accelerate their development in a safe, responsible way. The evidence base was covered from two angles:
- State of the Nation Survey which ran for four weeks between May and June of 2019 to build up a picture of critical issues surrounding ethics and regulation
- NIHR Innovation Observatory international horizon scan on the available evidence from academic publications, market authorisation and clinical trials databases.
The results of the 2019 Survey and NIHR horizon scanning exercise reinforced the 2018 survey results published in Accelerating Artificial Intelligence in Health and Care- Results from a State of the Nation Survey - that AI in health is in the early stage of the Gartner Hype Cycle. While significant progress has been made over the last year, just under half of the products available globally have market authorisation and just one third of AI developers in the UK believe that their product will be ready for deployment at scale in one year (as shown below).
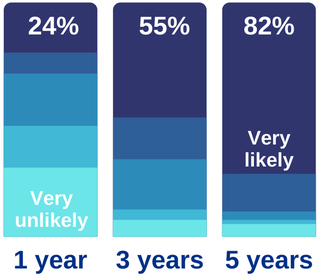
In addition to low market-readiness, the results also show that interest from AI developers is not yet evenly spread across all opportunity areas for AI-Health. The results of the Horizon Scanning exercise show that diagnosis and screening are the most common uses of AI, with 132 different AI products used in diagnosis or screening covering 70 different conditions. Of these, 90 products addressed priorities in the Long-Term Plan and, within these, 45 had European market authorisation. Based on this analysis, interpretation of images in screening mammography, retinal imaging, X-Ray, cardiac monitoring and head CT appear to be the areas with the greatest development activity
Areas of greatest development in AI or data-driven technologies
- Heart health and atrial fibrillation: 8 products
- Intracranial imaging/diagnosis of stroke: 7 products
- Breast cancer imaging: 5 products
- Chest X-ray interpretation: 3 products
- Retinal imaging: 2 products
- Use of AI (from the "State of the Nation" survey): 48.15% diagnosis; 51.85% other (e.g. self care and monitoring)
- Number of unique products (from the NIHR Innovation Observatory): 137 products
- Number of products that meet NHS Long Term Plan priorities: 90
- Number of products with EU marketing authorisation: 40
AI can be used more readily in diagnostics for two main reasons:
- Most radiology images are in a standardised digital format i.e. they provide structured input data for training purposes, compared to the unstructured and often non-digital data of health records, for example. This also means there are good data sets available for retrospective algorithm training and performance validation.
- Image recognition machine learning techniques are more mature. The evidence so far shows that algorithms can, within constrained conditions, be used to identify the presence of malignant tumours in images of breasts (7,8) , lungs (9) , skin (10), and brain (11) as well as pathologies of the eye (12) to name a few.
As images are typically produced and evaluated in hospital settings by clinicians, this could explain why the survey showed 67% of all solutions are currently being developed for use by clinicians and 59% are designed to be deployed in secondary care settings.
This is higher in the case of diagnostics specifically, in which 83% of solutions are being developed for use by clinicians and 73% for use in secondary care.
The purpose of many of these diagnostic-specific solutions is to speed up the rate of diagnosis and/or to identify the patients most at risk (so they can be prioritised) as well as to help the NHS cope with staff shortages by making more effective use of the radiologists available. This is reinforced by the survey findings which show solutions are being developed to achieve quicker diagnosis (79%), faster identification of care need (63%), and better experience of health services (63%). Overall, 71% of diagnostic solutions are designed to deliver on the outcome of ‘system efficiency’.
These are exciting results. However, before the NHS can capitalise on these opportunities, the ecosystem as a whole (e.g. developers, regulators, innovators, policymakers etc.) needs to consider:
- How to validate the results of individual studies – to check, for example, whether the algorithm is equally capable of recognising malignancy in mammography scans of people with different ethnicities.
- How to model the impact on individual pathways and the system as a whole. For example, we need to assess whether speeding up the rate at which people are ‘diagnosed’ could lead to longer anxious waits for treatment if the capacity of the system to treat is not increased as well.
- How to ensure consistently good public engagement with the concept of AI as a whole and with specific technologies.
In addition, the results show that more work is needed to ensure the datasets vital to the development of life-saving AI technologies are FAIR (findable, accessible, interoperable, and reusable)13 and used appropriately. As whilst the results show that in almost all instances the responses to the question on ‘provider of data’ and ‘data controller’ remain the same, and there has been some investment in the development of sophisticated modelling techniques.
For example, 19% of solutions are being developed on algorithmically generated datasets and this is likely to increase. However, the majority (57%) are still reliant on patient data either provided by Acute Hospital Trusts (55%) or patients themselves (23%) through the use of third-party apps. Furthermore, most developers were unaware of the commercial arrangement they had in place to gain access to the data.
Are developers seeking ethical approval?
It is currently quite hit and miss whether or not developers seek ethical approval at the beginning of the development process with an almost 50/50 split between those that did and those that did not.
This shows how the current complex governance framework for AI technologies is perhaps limiting innovation and potentially risking patient safety. The survey results also reveal that it is currently quite hit and miss whether or not developers seek ethical approval at the beginning of the development process with an almost 50/50 split between those that did and those that did not. This is in part due to a lack of awareness: almost a third of respondents said they were either not developing in line with the Code of Conduct or were not sure. The main reason given for this was ‘I was unaware that it existed.’ We (NHSX) will need to ensure that in all funding applications the expectation of compliance is made clear.
Similarly, the survey indicates that half of all developers are not intending to seek CE Mark classification (ie, they are not intending their innovation to become certified as a medical device). The reason most commonly cited was that the medical device classification is not applicable. This may be the result of a general misunderstanding as it is unclear in many cases whether or not ‘algorithms’ count as medical devices. This lack of certainty may even increase with new guidance coming into force in May 2020 and May 2022.
A greater degree of clarity is required regarding the regulator requirements for ‘real AI.’ The impact of this lack of clarity is obvious, with some companies developing technologies without (or at least not earlier enough) consideration of issues such as bias, discriminatory outcomes and explainability (see the survey results below).
Percentage of developers considering ethical issues
Results from the "State of the Nation" survey.
Have you assessed possible issues of bias in your data samples?
Yes: 81%; No: 14%; Don't know: 17%
Have you considered whether your algorithmic system is fair and non-discriminatory in its architecture, procedures, and outcomes?
Yes: 84%; No: 13%; Don't know: 15%
Have you incorporated the explainability of the system into its design?
Yes: 83%; No: 9%; Don't know: 19%
Are you setting up procedures to make the rationale of the outputs of your system understandable to all affected stakeholders?
Yes: 96%; No: 5%; Don't know: 11%
Do you intend to seek access to separate datasets for testing purposes?
Yes: 71%; No: 23%; Don't know: 18%
Do you intend to seek access to separate datasets for validation purposes?
Yes: 76%; No: 21%; Don't know: 15%
Taken together, these results provide insight into the pain points experienced by innovators which NHSX and other partners will seek to address through the NHS AI Lab by:
- Further developing the Governance (ethics and regulation) Framework
- Providing more clarity around data access and governance
- Supporting the spread of ‘good’ innovation & monitoring its impact
- Upskilling the workforce
- Developing International Best Practice Guidance.
Algorithmically generated datasets
19% of AI solutions are being developed on algorithmically generated datasets and this is likely to increase
Developing a governance framework
(Jessica Morley, Caio C. V. Machado, Dr Christopher Burr, Josh Cowls, Dr Mariarosaria Taddeo & Prof Luciano Floridi)
Why you need ethics and regulation
In delivering on the aim of NHSX to create an ecosystem that ensures we get the use of Artificial Intelligence ‘right’ in health and care we need to be aware of:
a) Generic data and digital health considerations:
i) Data Sharing & Privacy (46–51)
ii) Secondary uses of healthcare data (29,52–54
iii) Surveillance, Nudging and Paternalism (55–58)
iv) Consent (15–18)
v) Definition of Health & Care Data (19–22)
vi) Ownership of Health & Care Data (15,23–26)
vii) Digital Divide/eHealth literacy (27,28)
viii) Patient Involvement (29,30)
ix) Patient Safety (31)
x) Evidence of Efficacy (32–34)
b) Specific Algorithmic Considerations (35):
i) Inconclusive, inscrutable or misguided evidence leading to e.g. misdiagnosis or missed diagnosis, de-personalisation of care, waste of funds or loss of trust (36–38)
ii) Transformative effects and unfair outcomes leading to e.g. deskilling of healthcare practitioners, undermining of consent practices, profiling and discrimination (22,39,40)
iii) Loss of oversight leading to e.g. lack of clarity over liability with regards to issues of safety and effectiveness (41–44) To ensure that these considerations do not hinder the development or deployment of AI technologies, we need to consider the ethical, regulatory and legal framework in addition to the technical possibilities and limitations and governance mechanisms currently in place (45).
It is important to have both ethical frameworks and appropriate proportionate regulations (covered in detail below) because regulations only tell those developing, deploying or using AI what can and cannot be done whilst addressing the important safety element. This is not sufficient cover in the sensitive areas of health and care when due consideration also needs to be given to whether something should or shouldn’t be done. This is why we also need soft ethics (46).
By considering the ethical implications, we can make sure that we develop frameworks that not only cover the intentions and responsibilities of different people involved in developing, deploying or using AI, but also the impacts that AI has on individuals, groups, systems, or whole populations. Ultimately, this means we can tackle any potential harms proactively rather than reactively (47).
A code of conduct
( Dr Indra Joshi and Jessica Morley)
The Code of Conduct for Data-Driven Health and Care Technology, initially published in September 2018 and since revised following extensive feedback, is a core resource for anyone involved in developing, deploying and using data-driven technologies in the NHS. It provides practical ‘how to’ guidance on all the issues surrounding regulation and access to data.
The Code has been recognised around the world as a leading source of guidance to ensure that AI is responsibly and safely used, and addresses the need for more agile regulation- that is safe, effective and proportionate- in an environment where the pace of innovation is always going to be quicker than the ability of regulatory authorities to keep up.
The Code aims to promote the development of AI in accordance with the Nuffield Council on Bioethics’ principles for data initiatives (i.e. respect for persons, respect for human rights, participation, accounting for decisions), and does this by clearly setting out the principle behaviours that the central governing organisations of the NHS expect, as follows:
- Understand users, their needs and the context.
- Define the outcome and how the technology will contribute to it.
- Use data that is in line with appropriate guidelines for the purpose for which it is being used.
- Be fair, transparent and accountable about what data is being used.
- Make use of open standards.
- Be transparent about the limitations of the data used.
- Show what type of algorithm is being developed, or deployed, the ethical examination of how the performance will be validated, and how it will be integrated into health and care provision.
- Generate evidence of effectiveness for the intended use and value for money.
- Make security integral to the design.
- Define the commercial strategy.
Most of these principles reflect behaviours that are already required by regulation, such as the Data Protection Act 2018, or existing NHS guidance, such as the NHS Digital Design Manual. Principles 7 and 8 and 10 are entirely new and required further supporting policy work.
Principle 7: Algorithmic explainability
(Olly Buston, Dr Matthew Fenech, Nike Strukelj, Areeq Chowdhury, Jessica Morley and Dr Indra Joshi)
Principle 7 on ‘algorithmic explainability’ aims to tackle the ‘black box’ nature of digital healthcare applications and provide clarity to patients, users, and regulators on the functionality of an algorithm, its strengths and limitations, its methodology, and the ethical implications which arise from its use. The principle is described in detail as: ‘show what type of algorithm is being developed, or deployed, the ethical examination of how the data is used, how its performance will be validated, and how it will be integrated into health and care provision.
To help developers with this principle, NHSX has been working with Future Advocacy and other partners (including academic, industry and patient groups), to create a ‘how to’ guide for developers. The guide takes the form of a set of processes (below) that NHSX will encourage developers to undertake.
The processes are divided into:
- recommendations for general processes that apply across all aspects of principle 7; and
- recommendations for specific processes that apply to certain subsections.
In both cases, the intention is to make it very clear to developers not only what is expected of them in order to develop AI for use in health and care, but also how they might go about doing it. This is because ethical and behavioural principles are necessary but not sufficient to ensure the design and practical implementation of responsible AI. The ultimate aim is to build transparency and trust.
5 components for stakeholder analysis
- Assess data issues and identify algorithm(s)
- Prove algorithm(s) is/are effective
- Consider interaction with wider healthcare system
- Comply with ‘right to an explanation’
- Explain how acceptable use of algorithm determined
Finally - include an open publication of results.
General process: Stakeholder analysis
Undertaking a robust and inclusive process of stakeholder analysis will help highlight and preserve relationships of importance in healthcare, ensuring that the various players in the diverse relationships making up a healthcare system are identified and involved in the development process.
This should go beyond simply identifying direct and indirect stakeholders and provide a deeper understanding of the wider cultural context (be it in the healthcare system or in wider society) in which the data-driven tool will be embedded. To ensure this output, stakeholder requirements and concerns (that is, both positively-valued and negatively-valued beliefs) should be considered through the use of value and consequence matrices (below). This process should be repeated at regular intervals.
a) Value matrix
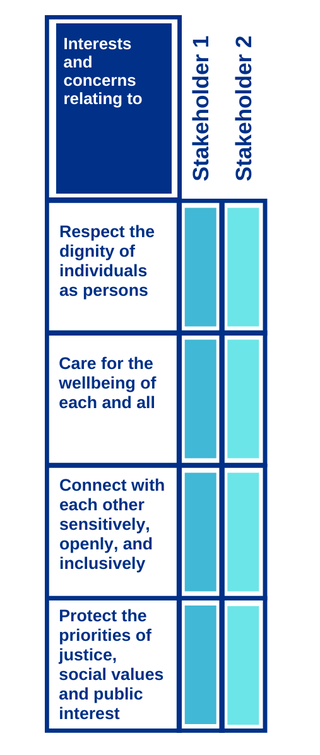
b) Consequence matrix

Specific Processes:
- Report on the kind of algorithm that is being developed/ deployed and how it was trained, and demonstrate that adequate care has been given to ethical considerations relating to the selection of, obtaining of, and use of data for the development of the algorithm.
- Reflect on the proposed means of collecting, storing, using and sharing data, and on the proposed way that their algorithm(s) will work by using ‘Datasheets for Datasets’ (49) or Open Data Institute’s ‘Data Ethics Canvas’ (50)
- Identify what type(s) of algorithm(s) constitute the data-driven technology, and answer specific associated questions with that type of algorithm. For machine learning models, developers could adopt the ‘model card’ approach (51)
2. Provide supportive evidence that the algorithm is effective :
- Submit the data-driven tools for external validation against standardised, validated datasets (as and when these become available)
- Engage with NHSX at the earliest stage of development, in order to communicate :
i. The proposed method of continuous audit.
ii. The expected inputs and outputs against which performance will be continuously audited.
iii.How these inputs and outputs were determined. iv. How these inputs and outputs are likely to impact the different stakeholders identified in the stakeholder analysis. - Use standard reporting frameworks, such as those being developed by the EQUATOR Network.
3. Demonstrate that due consideration has been given to how the algorithm will fit into the wider healthcare system, and report on potential wider resource implications of deployment of the algorithm:
- Identify :
i. The need/use case for the data-driven technology, and the existing care pathway(s) impacted by the tool.
ii. The associated care pathways that interact with the target care pathway. For example, a tool designed for patients with diabetes may well have impacts on cardiovascular disease care pathways, and renal disease care pathways, as patients with diabetes are frequently seen on these pathways.
iii.The potential impacts on these target and associated care pathways of the tool.
4. Explain the algorithm to those taking actions based on its outputs, and to those on the receiving end of such decision-making processes:
- Clarify the extent to which a decision based on an algorithmic tool is automated and the extent that a human has been involved in the process—that is, full transparency on the use of an algorithm.
- Use the stakeholder analysis exercise to clarify what is meant by the term ‘meaningful explanation’ for each stakeholder group.
- Coordinate with patient representative groups and other stakeholders to help develop ‘meaningful’ language as part of the explanation that will be understood by patients and other stakeholders.
- Where explanations remain too complex for lay comprehension, developers should support third parties that are trusted by patients (e.g. disease-specific charities) in acting as advocates for their patient groups.
5. Explain how the decision has been made on the acceptable use of the algorithm in the context it is being used (i.e. is there a committee, evidence or equivalent that has contributed to this decision):
- Utilise specifically-designed activities (such as user research, talking to patient groups and representatives, citizen juries, etc) to assess thinking on the acceptable use of an algorithm. For example, nurses and clinicians should participate in the development of an algorithm that determines staff rotas.
- Openly document the justification for and planning of these activities .
- Monitor user reactions to the use of the data-driven technology, and gauge levels of its acceptance on a recurrent basis.
Principle 8: Evidence for Effectiveness
(Mark Salmon, Bernice Dillon, Indra Joshi, Felix Greaves and Neelam Patel)
For principle 8, which is to ‘generate evidence of effectiveness for the intended use and value for money’, NHSX worked with the National Institute for Health and Care Excellence (NICE), Public Health England (PHE), and MedCity (the life sciences sector cluster organisation for the Greater South East of England), to create the Evidence Standards Framework for Digital Health Technologies .
The framework establishes the evidence of effectiveness and economic impact required before digital health interventions can be deemed appropriate for adoption by the health and care system. In keeping with its principled proportionate approach, the framework is based on a hierarchical classification determined by the functionality (and associated risk) of the tool, which indicates the level of evidence required; for example, a more complex tool (such as one providing diagnosis) requires considerably more evidence than one simply communicating information.
The framework is important to encourage adoption of new technologies, including AI, as it is vital that those using them in the provision of care are confident that they work safely. For the NHS as a whole, it is also important that the cost and effectiveness of using a specific technology over another or a non-technological solution can be justified. In the traditional practice of evidence-based medicine this evidence is generated by randomised controlled trials. This is not always practical for digital health technologies (DHTS) or AI so NICE published the evidence standards framework for DHTs, with supporting case studies and educational materials, in March 2019.
The standards in the framework cover evidence of clinical effectiveness and economic impact and provide a common reference standard for discussions between innovators, investors and commissioners. They are designed to allow innovators to produce evidence that is better, faster, and at a lower cost and in turn; they also allow the NHS over time to commission and efficiently deploy (at scale), digital health tools that meet patient/ NHS need. Importantly, the evaluation of digital health and AI solutions can be standardised, and is a key benefit.
Encouraging adoption of new technologies
The framework is important to encourage adoption of new technologies, including AI, as it is vital that those using them in the provision of care are confident that they work safely
The framework may be used with DHTs that incorporate artificial intelligence using fixed algorithms. However, it is not designed for use with DHTs that incorporate artificial intelligence using adaptive algorithms (that is, algorithms which continually and automatically change). Separate standards (including principle 7 described in previous section) will apply to these DHTs.
What’s Next
To further build on this work, NICE is planning to set up a pilot DHT evaluation programme to establish a robust process for the national evaluation of these technologies. The aim is to enable NICE to issue positive recommendations to the NHS and care system for DHTs that offer a real benefit to patients and the NHS and social care systems.
The technologies being evaluated in the pilot will mainly be Tier 3b DHTs as defined in the NICE standards framework. These are the technologies that have measurable patient benefits, including tools used for treatment and diagnosis, as well as those influencing clinical management through active monitoring or calculation. The technologies incorporate AI to different degrees and include: a clinical decision support tool for triaging people for dementia assessment; a vital signs monitoring technology based on skin colour changes; and a technology for identifying cardiac arrhythmias.
The evaluation of the technologies will be based on the established medical technologies guidance development process and methods. However, for the pilot digital technologies this will be supplemented with a technical assessment which will include examining the extent of the use of AI . The review of the clinical evidence and economic impact will align with the NICE standards framework and so a key component of the pilot will be to explore how NICE can clearly specify the data that is required to address uncertainties in the evidence as early as possible to feed this into further development of the standards.
Principle 10: Commercial strategy
(Office of Life Sciences)
For Principle 10, described as ‘define a commercial strategy’, a set of additional principles were developed by the Office for Life Sciences to help the NHS realise benefits for patients and the public where the NHS shares data with researchers.
The aim of the principles is to help the NHS adapt to the ever-increasing need to share data between different parts of the healthcare system and with the research and private sectors to tackle serious healthcare problems through data-driven innovation. At the same time there is a need to put in place appropriate policies and delivery structures to ensure the NHS and patients receive a fair share of the benefits, and no more than their fair share of risk, when health data is made available for purposes beyond direct individual care.
As the technologies develop, the potential benefits and risks will shift, and so will the principles. As the frameworks iterate, it is crucial that the public feel as though they have been involved in the process. This is why NHS England and Understanding Patient Data are currently conducting research, based on public engagement and deliberation, to answer the question: what constitutes a fair partnership between the NHS and researchers, charities and industry on uses of NHS data (patient and operational)? Findings from this work will inform policy development being led by the Office for Life Sciences (OLS) - and will guide development of commercial model templates under the guidance of the National Centre for Expertise.
Self-assurance portal
(Jessica Morley, Sile Herz, MarieAnne Demestihas, Joseph Connor, Hugh Hathaway and Francesco Stefani)
NHSX is currently working with UCL to develop an online ‘Self-Assurance Portal’ to facilitate compliance with the Code of Conduct. The portal will help developers understand what is expected of them, prompting them to provide specific evidence for each principle. In this way NHSX hopes to not just be telling people what to do in order to develop responsible AI, but asking them to tell us how they did.
The portal is an online workbook version of the Code. Developers answer questions linked to each of the principles in the Code for each new product. For example, in relation to Principle 3, this question is asked: Is data gathered in the solution used solely for the purpose for which it is gathered? When users have provided responses to each set of maro-questions, they can see how their answers compare relative to others through visualisations.
Mapping the regulation journey
(Eleonora Harwich and Claudia Martinez)
Regulation is often perceived as being a barrier to the implementation and adoption of AI in healthcare. However, a closer look at the regulatory landscape shows that there are few issues with the regulation itself. The issues lie rather in the lack of coordination between regulators and statutory bodies along the innovation pathway[1]. In addition, the absence of a guidance and regulation navigator makes it difficult for people to figure out what they need to do and with whom they need to interact with at each stage of the process.
The journey map below provides a summary of a larger scale map looking at the regulatory landscape for data-driven technologies in England, from idea generation to post-market surveillance.
Broadly speaking, there are five types of pain points in the regulatory process (59). First, in the current landscape no one body/ unit is responsible for the overall process making it difficult to ensure coordination between regulators. Second, regulation can often be wrongly interpreted on the ground, particularly regarding regulation around data. Third, in some very specific instances, the regulation itself is not fit-for-purpose. The letter of the law would require people to go through such cumbersome processes that regulators follow the ‘spirit of the law’ instead. Fourth, in some cases the remit of regulators is unclear or overlapping, which means that no one is responsible for policing a specific regulatory requirement. No regulator has direct oversight over the quality of the data used to train algorithms, meaning that no one is responsible for preventing bias in algorithmic tools. Finally, there are uncertainties about how to regulate certain aspects of AI.
There are five regulators involved in the regulation of data-driven technologies in healthcare: the Care Quality Commission, the Information Commissioner’s Office, the General Medical Council, the Health Research Authority and the Medicines and Healthcare products Regulatory Agency. Another four statutory bodies: the National Data Guardians, NHS Digital, NHS England & Improvement and the National Institute for Health and Care Excellence. There is also a multitude of other bodies with a role in this field. This larger scale map was produced thanks to a thorough literature review, 40 semi-structured interviews and three workshops with a total of 31 participants.
Current regulator journey map for data driven technologies in health and care
Data access journey map
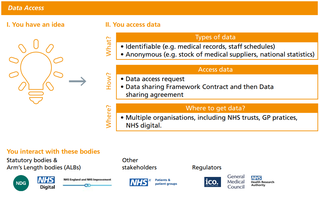
Proof of concept journey map
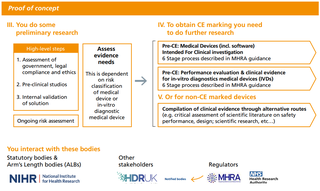
Regulatory compliance journey map
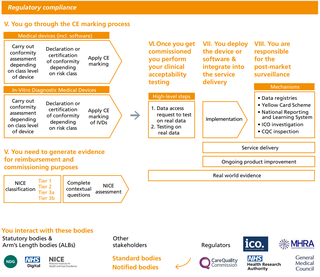
The data access stage
The data access stage causes a lot of confusion. People have difficulties in determining the legal basis for processing data and if their project should be classed as research or not.
The data access stage causes a lot of confusion. People have difficulties in determining the legal basis for processing data (i.e. direct patient care or secondary uses) and if their project should be classed as research or not. For instance, developing a piece of software using medical data should always be considered as a secondary use regardless of that software eventually being used to provide direct care to the patient. It should also be classed as research and obtain approval from the Health Research Authority (HRA) (60).
The proof-of-concept stage helps to assess the feasibility and practical implementation of data-driven technologies. At this stage, manufacturers might conduct pre-clinical studies or academic research as well as test the validity of algorithms. Manufacturers will also start generating the clinical and technical evidence required to obtain the CE marking and for getting their product commissioned by the NHS. Existing regulation and the Medicines and Healthcare Products Regulatory Agency’s (MHRA) guidance on CE marking for medical devices and in-vitro diagnostic medical devices is clear and accessible61. Confusion exists, however, regarding the type and routes to obtaining evidence for products not undergoing a CE marking procedure.
The regulatory compliance stage is relatively straightforward as the MHRA’s guidance is clear (62). At this point, innovators would also have engaged with the notified bodies who carry out the conformity assessments. There are nevertheless regulatory challenges which particularly affect the regulation of AI. For example, there are no standards in place for the validation of algorithms, although, there is currently a project looking into this issue between NHS Digital and the MHRA (63). There is also a lack of clarity about regulating ‘adaptive’ algorithms and its implications for regulatory compliance.
Overcoming regulatory pain points
Overcoming the pain points in the development pathway will be a priority for the NHS AI Lab. This will be a long-term and evolving project and guidance will need to adapt as technologies develop. There are a number of projects already underway to get the process started, and include:
- Work by the Care Quality Commission to develop principles for encouraging digital innovation as part of the ‘well led’ criteria of assessments.
- Work by NHS England to update the NHS Code of Confidentiality to ensure that it enables research.
- Development of HealthTech Connect by NICE:
a. Companies (health technology developers or those working on behalf of a health technology developer) can register with www.HealthTechConnect.org.uk. Data can be entered and updated about a technology as it develops. It is free of charge for companies to use.
b. The system will help companies to understand what information is needed by decision makers in the UK health and care system (such as levels of evidence), and clarify possible routes to market access.
c. The information entered will be used to identify if the technology is suitable for consideration by an organisation that offers support to health technology developers (for example, through funding, technology development, generating evidence, market access, reimbursement or adoption).
d. It will also be used to identify if the technology is suitable for evaluation by a UK health technology assessment programme.
e. Technologies that are suitable for support or evaluation will be able to access it through HealthTech Connect. This will avoid the need for companies to provide similar, separate information about the technology to the organisation or programme. - Development of synthetic datasets by MHRA and NHS Digital (as referenced above).
- Research by the Information Commissioner’s Office and the Alan Turing Institute as part of Project ExplAIn to create practical guidance to assist organisations with explaining artificial intelligence (AI) decisions to the individuals affected.
- Launch of the Care Quality Commission’s regulatory sandbox for health and social care. The sandbox is running a cohort specifically for machine learning and its application to Radiology, Pathology, imaging and physiological measurement services. They will focus on developing their registration and inspection policies with industry and NHS partners. CQC are also partnering with the MHRA, British Standards Institute, NICE, and NHSx as part of this round to consider the gaps and overlaps with other regulators, and the wider issues around adopting these technologies in clinical practice.
These programmes of work have put solid foundations in place, but by creating the NHS AI Lab, and investing significantly in the development of both the regulatory frameworks themselves and the technical techniques to ensure compliance, the UK can deliver on its promise to be the best place to practice responsible AI.
Clarifying data access and protection
(Dawn Monaghan and Juliet Tizzard)
Navigating data regulation
As highlighted in the previous section, access to health data is necessarily complex as it requires safeguards, high levels of security and data minimisation to mitigate the risk of re-identification and clear controls to ensure it is used only for appropriate purposes.
As a result, Information Governance is often cited as a problem when attempting to introduce new technologies or ways of working. This is particularly the case if the application of the rules is misunderstood or the interpretation of the rules has been turned into a complex ‘black art’.
However, it’s important to realise that the legal obligations of Data Protection are meant to enhance the processing of information. They can facilitate the ability to meet business needs whilst protecting individuals’ privacy and confidentiality and upholding their rights.
Importantly, the principle of data minimisation is a key concept which must be embraced.
The first questions to ask are, what data is actually required and can the aims be achieved by using data other than Confidential Patient information?
- Identifiable data – In law it has different names - Personal Data or Confidential Patient Information - with different definitions but it includes data where an individual can be identified either directly from the dataset or in combination with other datasets.
- Anonymous data – Data in a form that does not identify individuals and where identification through its combination with other data is not likely to take place.
- Synthetic data – A synthesised, representative dataset which does not relate to any real people.
If Confidential Patient Information needs to be used then the full remit of the IG principles need to be adhered to and ethical considerations taken into account.
Completing a DPIA
Completing a Data Protection Impact Assessment (DPIA) will help capture potential impacts, consider mitigations and enable informed risk management and proportionality judgements to be taken.
Applying the notion of ‘Privacy by Design’ at the concept stage can identify possible impacts that the proposed product or way of working may have on an individual’s privacy and will help assure legal compliance and maintain trust. Completing a Data Protection Impact Assessment will help capture potential impacts, consider mitigations and enable informed risk management and proportionality judgements to be taken. It should be part of the business governance process.
In essence when considering the IG implications there is a need to be clear about:
- What data is required.
- Why is it required.
- Who will be processing it (will it be shared).
- How will it processed (including security, storage, etc).
- Where will it be processed.
Once the above information is articulated an impact assessment can be undertaken and safeguards put in place to meet key principles and remain compliant.
Whilst lawfulness must be paramount, the requirements of not only the Data Protection Act but other relevant legislation such as the Common Law Duty of Confidentiality must be adhered to. However, other principles are just as important, and none more so than the need for transparency. It is essential that any use of data is transparent; this not only secures compliance but ensures that citizens’ expectations are taken into account and managed, with public trust maintained.
When using techniques such as AI the impacts on individuals need to be fully explored, particularly if decisions may be taken without any human involvement. There are specific rules which apply to automated processing to ensure individuals understand the process and how it can affect them.
However, if the appropriate approvals are sought and the proper protections are in place, then it is important to facilitate access to it so that the many benefits of AI and other data-driven technologies can be unlocked. This is why we are investing in a number of important projects designed to facilitate access for the purpose of delivering better health outcomes.
Understanding patient data
(Dr Natalie Banner)
Many people are not aware that the information within their health records has enormous research potential, nor how it could actually be used in practice or what their choices are. Unsurprisingly, there are significant public concerns especially when commercial organisations may be involved in data use.
Understanding Patient Data (UPD) exists to try and help make the uses of patient data more visible understandable and trustworthy, for patients, the public and health professionals alike. We work with charities, patient groups, academic researchers, healthcare providers, media, data custodians and policy makers to champion responsible uses of health data. Data is a complex and technical landscape, so we produce freely available resources that seek to demystify health data use. This includes guidance on jargon-free language, and animations that tell stories about patient data in an engaging way. We also bring together networks of advocates and provide a unified voice to raise concerns or challenges to policymakers about the rules, transparency and accountability of health data use.
With the advent of data-driven technologies, it will be more important than ever to find creative ways both to inform people about health data use, and to involve those who are interested or concerned in governance and decision-making at local and national levels, to ensure the systems for managing and making use of the data are worthy of the trust people are being asked to place in them.
UPD was set up as an independent initiative in 2016 partially in response to the National Data Guardian’s call for a better public conversation about health data. It is primarily funded by Wellcome, a global charitable foundation dedicated to improving health.
The National Data Guardian
The National Data Guardian works to ensure citizens’ confidential information is kept safe and confidential, and that it is shared when appropriate to achieve better outcomes for patients.
Protecting the citizen
The National Data Guardian (NDG) was created in November 2014 to be an independent champion for patients and the public. It works to ensure citizens’ confidential information is kept safe and confidential, and that it is shared when appropriate to achieve better outcomes for patients. The NDG does so by offering advice, guidance and encouragement to the health and care system.
The Health and Social Care (National Data Guardian) Act 2018 granted the NDG the power to issue official guidance about the use of health and adult social care data in England. This means that public bodies such as hospitals and GPs and private companies or charities that are delivering services for the NHS or publicly funded adult social care will have to take note of relevant guidance from the NDG.
The NDG wants to build trust in the use of data across the health and social care system and is guided by three main principles. First, to encourage clinicians and other members of care teams to share information that directly affects the care of the person they are treating or supporting. This can bring direct benefits to people such as joined-up care and better diagnosis and treatment. Second, to inform and provide citizens’ voice in how their health and care data is used. Third, to build a dialogue with the public, commercial companies, researchers and service providers about how information should be used.
The NDG Panel is a group of experts appointed by the NDG to advise and support its work to represent the interests of patients and the public. The UK Caldicott Guardian Council is a sub-group of the NDG Panel and is responsible for protecting the confidentiality of people’s health and care data and ensuring that it is used properly. All NHS organisations and local authorities that provide social services must have a Caldicott Guardian.
Data innovation hubs
(Caroline Cake, Health Data Research UK)
The Digital Innovation Hub Programme managed by Health Data Research UK (Health Data Research UK) aims to become a UK-wide life sciences ecosystem providing responsible and safe access to health data, technology and science, and research and innovation services to ask and answer important health and care questions.
This four-year programme is funded by the UK Research and Innovation’s Industrial Strategy Challenge Fund (ISCF). The programme integrates with and reinforces Health Data Research UK’s investment in health data science and talent development, to stimulate the use of health data to make lives longer and healthier. It encompasses three essential functions:
- UK Health Data Research Alliance – an Alliance of data custodians committed to making an unprecedented breadth and depth of data available for research and innovation purposes for public benefit.
- Health Data Research Hubs – making data available, curating data, and providing expert research services. The Hubs will be centres of expertise to get from raw and fragmented data to insight and the location to collaborate and co-create.
- Health Data Research Innovation Gateway– providing discovery, accessibility, security and interoperability to surface data, support linkage, and enable health data science safely and efficiently.
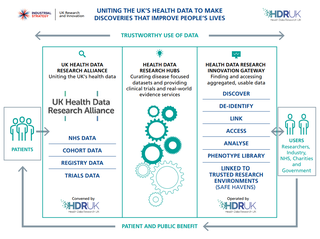
The NHS AI Lab working in partnership with Health Data Research UK through the UK Health Data Research Alliance will help create the pipeline from development to deployment of AI systems within health and care.
Data collaboration at scale
The UK Health Data Research Alliance is an independent, not-for-profit alliance of health data custodians from the UK’s leading health and research organisations [members] united to establish best practice for the ethical use of UK health data for research at scale.
75% of Global Digital Health Partnership members say...
75% of Global Digital Health Partnership members say that the body (or bodies) responsible for regulating digital health technologies is currently looking to change their remit and adapt regulations appropriately
The UK has some of the richest health and research datasets and assets world-wide. Some of these are well organised, but only a fraction of all NHS and research data is accessible. By developing and co-ordinating the adoption of tools, techniques, conventions, technologies, and designs, the Alliance enables the use of health data in a trustworthy and ethical way for research and innovation.
For researchers and innovators to benefit from secure access to an array of health-related data and address health problems, we need the right expertise, trusted governance, and collaboration through a UK-wide research alliance. The UK Health Data Research Alliance is coordinated by Health Data Research UK and was formally launched on 7 February 2019. Other custodians of large-scale health data are warmly welcomed to join the Alliance to widen the opportunities for medical breakthroughs.
Data agreements and commercial models
Data sharing agreements and ‘future fit’ commercial model templates will address a clear pain point for innovators. Questions on data access and sharing are complex and will have different answers in different contexts. A key challenge is answering when, by whom, why, where and how data (especially sensitive data) can be accessed (16). Commercial models will engender trust, reduce negotiation time and complexity, and help innovators meet Principle 10 of the Code of Conduct.
This is why in addition to guidance provided by the Code of Conduct, The National Data Guardian, The Information Commissioner’s Office, and the guiding principles in the framework for data sharing with researchers published in July 2019, NHSX is committed to developing a National Centre of Expertise to oversee the policy framework, provide specialist commercial and legal advice to NHS organisations entering data agreements, develop standard contracts and guidance, and ensure that the advantages of scale in the NHS can deliver benefits for patients and the NHS.
The National Centre of Expertise will have a crucial role to play in ensuring that data sharing agreements meet the first guiding principle that “any use of NHS data, including operational data, not available in the public domain must have an explicit aim to improve the health, welfare and/or care of patients in the NHS, or the operation of the NHS.” (31)
The Centre of Expertise will sit in NHSX and its core functions will include:
- Providing hands-on commercial and legal expertise to NHS organisations – for potential agreements involving one or many NHS organisations (eg for cross-trust data agreements or those involving national datasets). This could include providing support to negotiate and execute agreements, and assessing and building capability within NHS organisations where useful. The Centre will develop and provide tailored legal advice on relevant issues (eg intellectual property, state aid).
- Providing tools and products including good practise guidance and examples, standard contracts, and methods for assessing the value of different partnership models to the NHS.
- Signposting NHS organisations to relevant expert sources of guidance and support on matters of ethics and public engagement, both within the NHS and beyond.
- Engagement and understanding the landscape – building relationships and credibility with the research and industry community, regulators, and with NHS organisations and patient organisations, including developing insight into demand for different datasets and identifying and communicating opportunities for agreements that support data-driven research and innovation.
- Developing benchmarks and scenarios to provide NHS organisations with reference points on what ‘good’ looks like in agreements involving their data, taking into account demand for data, market conditions and the international context, and setting clear and robust standards on transparency and reporting to underpin and support public trust.
NHSX data framework
NHSX is committed to creating an environment that will facilitate access to data, by reducing barriers and delays to data access and providing clarity to both data owners and companies on how to help the process run smoothly. A Data Framework was published in July 2019 that sets out five guiding principles to maximise benefits for patients and the public where the NHS shares data, and which will underpin successful innovation in the NHS AI Lab.
Ongoing work by the NHSX team in the mandating of interoperability standards, by NHS England in the development of programmes such as the Local Integrated Health and Care Record Exemplars, and Health Data Research UK in providing a single point of access and facilitating the development of Digital Innovation Hubs (as well as others), will be enabled through this framework.
Encouraging spread of "good" innovation and monitoring the impact
What does good AI look like?
1. Precision medicine
(Professor Philip Beales)
Precision medicine encompasses predictive, preventive, personalised and participatory medicine (also termed P4 medicine)17. It is moving from the traditional one-size-fits-all form of medicine to more preventative, personalised, data-driven disease management model that achieves improved patient outcomes and more cost-efficiencies. Precision medicine, as defined by the National Institute of Health (NIH), is an emerging approach for disease treatment and prevention that considers individual variability in genes, environment, and lifestyle (18). The promise is that precision medicine will more accurately predict which treatment and prevention strategies will work best for a particular patient.
In the broader setting, AI is helping industry to accelerate drug development, cut costs and gain faster approvals while reducing errors. Data-driven health will also likely impact patients directly by providing access to their own personal data, improving compliance with treatments and real time monitoring for adverse events making participation in P4 medicine a reality.
2. Genomics
(Anna Tomlinson, Chris Wigley, MJ Caulfield and John Hatwell)
Key to unlocking the benefits of precision medicine with AI is the use of genomic data generated by genome sequencing. Machine learning is already being used to automate genome quality control. AI has improved the ability to process genomes rapidly and to high standards and can also now help improve genome interpretation.
Genomics England, which currently contains over 100,000 genomes and over 2.5 billion clinical data points, has developed a platform to capture the substantial amounts of data from automated genome sequencing and healthcare professionals that AI needs.
Researchers are using machine learning methods to identify genetic mutation signatures associated with certain cancers, for example, helping to identify and classify sub-types of the disease and to identify targets for new treatments.
Whilst AI will undoubtedly accelerate progress, we should be careful to supervise machine learning and people are still needed to avoid misinterpretation of data in making care decisions. In order to build an optimal and sustainable future for genomic medicine, earning, building and retaining trust with patients, the public and technology and research partners, will be essential.
3. Image recognition
(Simon Harris and Deirdre Dinneen)
The East Midlands Radiology Consortium (EMRAD) is a pioneering digital radiology system, and is a partnership of seven NHS trusts[i] spread over 11 hospitals, covering more than five million patients. The cloud-based image-sharing system has set the national benchmark for a new model of clinical collaboration within radiology services in the NHS.
In 2018, EMRAD formed a partnership with two UK-based Artificial Intelligence (AI) companies, Faculty and Kheiron Medical Technologies, to help develop, test and - ultimately - deploy AI tools in the breast cancer screening programme in the East Midlands. It aims to improve and optimise clinical service capacity, to enhance patient care at significant scale and to increase NHS confidence in the utilisation of innovative machine learning tools. The project aims to develop and test both clinical and non-clinical (operational) AI tools.
Kheiron’s Mia(TM) tool has the potential to support the clinical workforce issues in the service by acting as the second reader in the dual-read mammography workflow, while Faculty’s ‘Platform’ software has the potential to help optimise operational processes such as clinic scheduling and staff resourcing.
4. Operational efficiency
(James Teo and Richard Dobson)
Kings College Hospital NHS Foundation Trust together with the NIHR BRC at the South London and Maudsley Hospital have developed an open-source real-time data warehousing tool called ‘CogStack’. CogStack meets the acute need for a more efficient way to clinical code to improve financial and operational efficiencies for providers throughout NHS. The team at King’s College Hospital NHS Foundation Trust and the South London and Maudsley Hospital tested CogStack for clinical coding in a fracture outpatient clinic setting to identify under-coding and was able to triple the depth of coding within a month (from ~10% of cases to 30% of cases having procedures recorded accurately). This translates to £1,260,000 of financial activity per annum even without the efficiency gains.
Using modern open-source natural language techniques exploited by companies such as Google and openAI, the team have developed further advanced prototype NLP algorithms for performing large-scale tagging of clinical text (Semantic EHR; MedCAT) with machine learning; this has the potential to code all clinical data in real-time with associated efficiency gains. This is already functioning and deployed in a large NHS Trust, and has been tested with a real-world NHS problem showing it has potential for substantial disruption of manual healthcare processes. CogStack has been adopted by the open source community with major contributions to the evolving codebase coming from partners including UCLH BRC and Health Data Research UK.
Tackling barriers to adoption
(Asheem Singh and Charlotte Holloway)
Innovation is not just a set of technologies but an environment and a culture. The RSA conducted a research study to understand the ‘human story’ behind the challenge to spread technology - particularly new and complex technology - through the NHS. Over the course of June and July 2019, the RSA conducted 12 in-depth interviews (according to Chatham House rules) with professionals developing, procuring and using data-driven technologies across the country to understand what prevents people from adopting new technologies in the NHS to gain insight into:
- Commissioner, clinician and patient interactions with radical technologies.
- Barriers to adoption and pain-points.
- Mechanisms that might help facilitate these interactions and overcome the barriers.
The results show that even in this relatively small set of conversations, there was striking convergence on what needed to be done to smooth the adoption of AI into the health system and create a genuine, human-centred culture around technological innovation in the NHS.
The macro-level factors identified as being essential to clinical adoption of AI are: patient acceptance, evidence and clinical champions. The specific recommendations are:
- Put in place a rigorous anti-bias test.
- Prove the benefits of AI by, for example deploying back-end operational solutions first.
- Model the impact on clinical workflow.
- Make provisions for the continuous upskilling of the workforce.
- Scale up our sandboxing and piloting initiatives.
Measuring impact
(Dr Sarah Deeny, Dr Hannah Knight, Dr Geraldine Clarke, Josh Keith and Dr Adam Steventon)
The NHS faces a huge challenge to select the right technologies for adoption, given that so many new technologies are being developed, all with uncertain impact (52). These decisions should be informed by robust modelling of the potential impact of new technologies on patients and the health care system before they are implemented. Producing this information, or providing data that would allow the NHS and those commissioning technology to produce it, will help developers demonstrate the requirements of principle 2 of the code of conduct: “Define the outcome and how the technology will contribute to it”(6).
It’s vital that new technology addresses the most critical problems facing the healthcare system and its patients, but the technology’s potential impact will depend on where in the clinical pathway it is implemented. For example, an algorithm designed to detect diseases from medical images could be used within community settings (to detect patients needing referral to specialist care) or within outpatient care (to assist specialists with diagnosis). These will have different impacts. The modelling process can borrow from simulations and assessment frameworks (for example, those created to evaluate the potential impact of vaccination or screening programmes) (53,54), and be developed further to consider the potential impact of new AI tools. Such models should be developed and shared widely in the NHS, using open source methods where possible, and using well-established parameters for assessment where practical (such as those developed by NICE for cost effectiveness) (55).
Track the impact
The NHS needs to track the impacts of new technology in real-world settings so that benefits can be identified and spread, and potential harms spotted quickly.
Real-world evaluation
While working out if the technology works in a controlled setting is one thing, effective real-world piloting and evaluation is required to understand the wider conditions necessary to ensure technologies can deliver the benefits they promise, and how this impact might vary in different parts of the NHS.
The NHS needs to track the impacts of new technology in realworld settings so that benefits can be identified and spread, and potential harms spotted quickly. Technology companies need this information too, so they can improve their products and services over time. Several dimensions are relevant here, including impacts on patient outcomes and the demand for care, the workforce and wider system.
Monitoring the implementation of AI is challenging (57). These are complex interventions with multiple components and aims. Impact will be shaped by the context in which they are implemented, and the technologies themselves are rapidly evolving. An agile and multidimensional approach is needed, which combines both quantitative and qualitative methods (image below) (58,56).
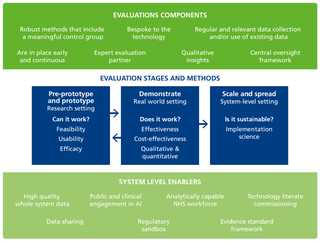
The impacts of new technologies will need to be assessed against a counterfactual, which charts the outcomes of existing forms of care for similar patients. This can often be achieved using existing NHS data, as demonstrated by the Health Foundation’s Improvement Analytics Unit (59). In some cases, NHS data will need to be combined with data from technology companies, which will require a data sharing agreement to be in place from the start (60).
Given the range of new technologies being developed, the NHS may need to invest in better analytical capability so that impacts can be assessed at local level (as outlined in the next chapter, on building capability and skills).
Creating the workforce of the future
(Professor Chris Holmes, Professor Sir Adrian Smith, Professor Andrew Morris, Dr Adam Steventon, and Ellen Coughlan)
The Topol Review published in February 2019 made recommendations that will enable NHS staff to make the most of innovative technologies such as genomics, digital medicine, artificial intelligence and robotics to improve services. The aim of the recommendations is to ensure the workforce is able to fully benefit from the introduction of AI into the NHS, enabling them to make the best use of their expertise, informing their decisions and saving them time.
As a practical example of these recommendations, the Alan Turing Institute, Health Data Research UK and the Health Foundation are exploring ways to work together to foster the development of analytical skills to enable the NHS to more readily take advantage of exponential developments in technology. Advances in algorithms, including AI tools, coupled with secure, scalable, computing environments that can access curated linked data will offer substantial opportunities for the NHS to improve patient outcomes and increase operational efficiencies. Certain core skill sets will be essential to meet this potential, enabling the design, build, and deployment of advanced analytics, all the while minimising financial, patient, and ethical risk.
A primary focus will be growing critical thinking expertise at all levels of NHS seniority to evaluate the potential for AI to benefit any given situation. This will include a technical understanding of requirements for the evidence base (the level and quality of curated data needed) and the computing resource needed to support both the design-deploy stage as well as the measurement and tracking of benefit. NHS staff need to be supported to understand the ethical considerations and regulatory procedures that are needed to sign off and monitor AI solutions.
Investing in the capabilities of NHS analysts is needed to enable them to develop the appropriate skills and understanding of the data in order to apply AI solutions effectively, and to interpret and communicate the complex information produced. The core analytical skills needed include:
1. an appreciation of how AI works, what its strengths and weaknesses are, and how it complements traditional statistical approaches;
2. the ability to design reproducible studies, using open-source analytics, built on an evidence base that is sufficient to learn from and to measure success on and;
3. an understanding of the protocols and meta-data needed to ensure robust execution of ethically fair solutions.
The NHS will also benefit from the creation of nimble tech teams, working hand-in-hand with data wranglers and external partners, focussed on rapid prototyping and evaluation of potential ideas.
The NHS can benefit from existing UK governmental and charitable investment in this space. Organisations like the Alan Turing Institute, Health Data Research UK (HDRUK) and the Health Foundation (HF) have significant experience in the provision of advanced analytic tools, the linking of health data at scale, and the appraisal and improvement of analytical capability in the UK’s health system[3]. These organisations have active training and development programmes that can support professionals from different backgrounds, and at all career stages, to use analysis and AI to improve patient outcomes and the efficiency of care. They are also working in partnership with NHS organisations to gather well curated data to improve the quality, safety and efficiency of care, underpin research, and generate new insights that can continuously improve decision-making by clinicians and patients.
Moreover, Turing’s embedded software engineering team, skilled in the prototyping and deployment of AI solutions, can help co-design, and share experience and technical insight into scalable algorithms and systems for learning. Ultimately, example programmes such as this underpins our ambition to ensure the people who keep the NHS running feel as supported as possible when delivering care, both now and in the future so that the NHS is the best place to work. This aim is a core part of the NHS Interim People Plan which reflects the beginning of a new way of working and supporting the NHS workforce to be the best it can be.
Developing international best practice guidance
(Clara Lubbers, Meredith Makeham, Rodney Ecclestone and Dr Indra Joshi)
The NHS AI Lab will be focused on making the UK a global leader in data and AI in the health and care space, and will be closely involved with key international initiatives as described below.
Global Digital Health Partnership
The Global Digital Health Partnership (GDHP) is a collaboration of governments and territories, government agencies and the World Health Organization, formed to support the effective implementation of digital health services. There are five key workstreams: 1) interoperability, 2) cyber security, 3) policy environments, 4) clinical and consumer engagement and 5) evidence and evaluation.
The GDHP aims to establish a baseline of activity and progress across GDHP participant countries and to examine practical approaches to the ethical, regulatory and legislative frameworks needed to enable data-driven technologies (for example, machine learning and artificial intelligence) to be successfully deployed in the delivery of health and care.
Machine learning and artificial intelligence have the potential to radically transform the delivery of health and care. However, the immense potential of these approaches requires digital health infrastructure that supports interoperability within and between countries to ensure its success. In this context, the GDHP seeks to assist policy makers to ensure that they are sufficiently aware of both the potential benefits and the potential issues to ensure they create an environment that maximises the chances of successfully achieving the benefits of emerging technologies. International collaboration in this area is providing governments with evidence to accelerate their approaches to local implementations and policy development relating to digital health technologies.
To help develop this international community, we carried out a survey and asked members a series of questions about their approaches to regulating AI, with an additional request for an example AI technology being developed in their country.
The aggregate results revealed that the development of AI on an international scale is out-pacing the development of the supporting policy framework. 62% of the AI solutions given as examples by members have already been deployed, yet less than half of all members (44%) have a national or regional policy framework for the development and/or deployment of AI.
Over 81% of members state that the national or regional body responsible for regulating digital health is currently not regulating adaptive algorithms in a clinical setting (none are regulating adaptive algorithms used in back-office settings), and 46% do not know whether the developers of the example provided sought ethical approval before development began. However, awareness of the need to develop this framework is growing.
75% of members confirm that the body (or bodies) responsible for regulating digital health technologies is currently looking to change their remit and adapt regulations appropriately.
World Health Organisation (WHO) & International Telecommunication Union (ITU)
(Sameer Pujari, Clayton Hamilton and Naomi Lee)
The World Health Organisation (WHO) in partnership with the International Telecommunication Union (ITU) has established a Focus Group on Artificial Intelligence for Health (FG-AI4H)61 with the aim of identifying opportunities for international standardisation and fostering the application of AI to health issues on a global scale. The work of the focus group encompasses development of a standardised assessment framework with open benchmarks for the evaluation of AI-based methods for health, such as AI-based diagnosis, triage or treatment decisions. The initiative represents a collective effort by the international community to systematically address the adoption of AI in health through a standardised and transparent evaluation of the underlying methods used.
44% of all GDHP members...
44% of all GDHP members have a national or regional policy framework for the development and/or deployment of AI
WHO’s actions for developing digital health are anchored in achievement of the UN Sustainable Development goals and in particular SDG target 3.8, the achievement of universal health coverage (UHC), which includes financial risk protection, access to quality essential health care services, and access to safe, effective, quality, and affordable essential medicines and vaccines for all.
Digital technologies, and AI in particular, are seen as having a key role in delivering on national policy through mechanisms to extend the scope, transparency and accessibility of health services and health information, hence reaching marginalised and underserved populations, and at the same time creating efficiency gains in the operation of health systems and improving the quality of care and treatment outcomes. To further guide the development of safe and inclusive digital health services in countries, WHO has been mandated to develop a Global Digital Health Strategy, which sets out a framework of action to advance and apply digital health towards achieving the vision of health for all.
Effective AI adoption requires us to identify potential health problems to which AI interventions can be assessed and applied. Rather than attempting to specify the AI for health algorithms themselves, or standardise across a multitude of medical data formats, the AI4Health focus group is working to establish a benchmarking framework, whereby eligibility of an AI model can be assessed, and feedback provided to improve quality.
This is being done by identifying common health domains (e.g. general diagnosis, specialty diagnosis, health natural language processing, general clinical encounter note data extraction and coding, Rx coding, lab coding, etc.) and for each domain to examine sourcing of test data, select current gold standard test success rates (e.g. how does a professional score on this test data), set benchmark rates for an AI system (to be acceptable for decision support, to be acceptable for autonomous operation), and define acceptable fail modes (e.g. alert human operator if below a given confidence threshold). As the work of the focus group progresses over time, several clinical and public health domains have emerged as priority areas for examination. These include cardiovascular disease risk prediction, diagnosis of bacterial infection and antimicrobial resistance (AMR), dermatology, falls among the elderly, histopathology, malaria detection and neuro-cognitive diseases.
Technical groups for Ethics and Regulation of AI have also been established under the Focus Group to examine existing approaches and provide standardised guidance for countries to follow.
Artificial Intelligence for Health also offers new ways to address the global shortage of healthcare professionals, which is a key issue decision makers face and one which threatens the sustainability of national health systems and health service delivery.
When implemented safely, AI has the potential to significantly improve and support medical diagnostics and treatment decision processes and strengthen the global public health role. It is hoped that the work of the WHO-ITU Focus Group in this area will accelerate adoption towards a safe and effective future of AI in Health.
The Equator Network
The EQUATOR (Enhancing the QUAlity and Transparency Of health Research) Network is an international initiative that seeks to improve the reliability and value of published health research literature by promoting transparent and accurate reporting and wider use of robust reporting guidelines.
To ensure transparency and reproducibility of any health intervention, whether it be a diagnostic test, a predictive model, an early warning system or a decision-making tool, complete reporting of study methods and results are of vital importance. The same principles apply to machine learning algorithms. Without complete and transparent reporting, it is difficult to assess the validity and generalisability of the study findings, which can result in misconceptions of overstated efficacy and utility of any health intervention. The risk is that an AI-intervention, which might not be effective or feasible in the real world, could be commissioned and implemented.
A recent systematic review (62) of over 20,000 studies evaluating the diagnostic accuracy of deep learning diagnostic algorithms across all medical domains highlighted a number of inadequacies in study reporting, including lack of clear details about the study population or datasets, the proportion and handling of missing data and full contingency tables (which allow the derivation of key performance metrics such as true positives, true negatives, false positives and false negatives). Only 82 studies made direct comparisons between deep learning algorithms and human healthcare professional accuracy, of which only 14 studies compare algorithms and humans using the same test validation dataset in an external validation. New reporting standards are under development to address these issues. The CONSORT-AI and SPIRIT-AI initiative (63,64) was announced in September 2019, to develop reporting guidance for studies evaluating AI interventions in clinical trials. Whilst such guidance is primarily for ensuring transparency of reporting, they can also be helpful for assisting clinical trial design.
Conclusion
The NHS AI Lab aims to bring together policies, partners and programmes to develop and deploy safe, effective artificial intelligence applications. The accelerating speed and complexity of applications means, however, that we need an agile, proportionate governance framework to harness the tremendous benefits of data-driven technologies while minimising the potential risks.
The Code of Conduct described in the report is a landmark step to this end, involving a number of partners working with NHSX to ensure the public is protected while benefiting from the exponential pace of developments we are seeing – addressing both the softer ethical considerations of the “should vs should not” in the development of AI solutions as well as the more legislative regulations of “could vs could not”.
Collaboration and partnership with organisations in a mutually - supporting ecosystem will become more and more important ahead, as the true potential of AI can only be realised through access to large volumes of FAIR data (findable, accessible, interoperable, and reusable) within an open environment to optimise research, testing and deployment at scale.
The future is exciting...
People will have better access to their own data which will help them to engage more in their own health.
An iterative, learning approach is also needed to develop the right skills and behaviours in the workforce to critically evaluate and develop AI applications that are inclusive, do not cause unintended harm and do not leave ‘anyone behind’.
The future is exciting – with opportunities that we can’t even imagine yet. People will have better access to their own data which will help them to engage more in their own health. Personalised medicine and predictive prevention will accelerate through greater access to clinical, genomic, phenotypic, behavioural and environmental datasets – where AI can spot patterns and opportunities that humans can’t. This will spur the continual development of novel breakthrough technologies and help the UK maintain and develop its position as a global leader in ethical AI.
Appendix: case studies
Flagship case studies
Precision medicine
(Professor Philip Beales)
Precision medicine encompasses predictive, preventive, personalised and participatory medicine (also termed P4 medicine) (17). It is moving from the traditional one-size-fits-all form of medicine to more preventative, personalised, data-driven disease management model that achieves improved patient outcomes and more cost-efficiencies. Precision medicine, as defined by the National Institute of Health (NIH), is an emerging approach for disease treatment and prevention that considers individual variability in genes, environment, and lifestyle18. The promise is that precision medicine will more accurately predict which treatment and prevention strategies will work best for a particular patient.
There are, however, significant challenges to the widespread adoption of precision medicine that include a deluge of medical data, a paucity of trained specialists, and the enormous costs of drug development19. Take, for example, the 30% rise in the number of CT scans ordered in the UK between 2013 and 201620 during which period the number of radiologists only increased by 3% annually (21). Many studies demonstrate that as radiologists are compelled to work faster their interpretation error rate rises (22) .
However, AI can address this by leveraging deep learning approaches to overcome the obstacles inherent in large data sets and unstructured data. In clinical settings, AI can assist clinicians to work more efficiently and make more accurate diagnoses improving the productivity of healthcare workers.
In the broader setting, AI is helping industry to accelerate drug development, cut costs and gain faster approvals while reducing errors. According to a recent research report (23), achieving the full potential of precision medicine will be impossible without applying AI and machine learning. Specifically, leveraging advanced machine learning and deep learning technology can outperform clinicians and researchers in rapidly analysing large datasets and integrating exponentially growing amounts of data from a wide variety of novel-omics sources (e.g. genomics, transcriptomics, proteomics, metabolomics, microbiomics) into clinically actionable insights for personalised care plans and more effective population health management.
One challenge is how best to integrate precision medicine data into electronic health records (EHR). OSF HealthCare, an integrated healthcare network, has integrated CancerIQ’s genetic cancer risk assessment program with Epic’s EHR platform as part of a systemwide population health initiative to reduce cancer disparities and deaths (24). In the US, individuals can now leverage Medfusion’s national health data network to consolidate their health records and help drive discovery through private, secure data sharing using LunaPBC part of LunaDNA, the first community-owned genomic and health data platform – that enables members to access their EHRs (25).
AI and machine learning are already transforming precision medicine delivery by driving computational phenotyping tools (www.mendelian.co) and new methods for target drug discovery (e.g. BenevolentAI). In fact there are now 100s of start-up companies using AI in drug discovery, (26) demonstrating the power of this emerging sector for driving innovation in healthcare.
In clinical trials, fewer than a third of all phase II compounds make it to phase III, and one-third of phase III trials fail because the trial lacks enough patients or the right kinds of patients. AI can potentially boost the success rate of clinical trials by identifying and characterising patient subpopulations best suited for specific drugs and by efficiently measuring biomarkers that reflect the effectiveness of the drug being tested. Nevertheless, we are still at the proof-of-concept stage but feasibility pilot studies are demonstrating the high potential of numerous AI techniques for improving the performance of clinical trials.
Data-driven health will also likely impact patients directly by providing access to their own personal data, improving compliance with treatments and real time monitoring for adverse events making participation in P4 medicine a reality
Genomics England
(Anna Tomlinson, Chris Wigley, MJ Caulfield and John Hatwell)
Key to unlocking the outlined benefits of precision medicine with AI, is the use of genomic data generated by genome sequencing. Genomics England has been using these immense datasets in the 100,000 Genomes Project to provide diagnoses and stratification to rare disease and cancer patients. However, they realise that this is still only scratching the surface of what genomics will be able to offer – it is fully expected that the use of AI based tools will dramatically accelerate progress in this field. As Genomics England expands its vision to sequence 5 million genomes over the coming years, these tools will become ever more important.
Machine learning is already being used to automate genome quality control. Using machine learning we can identify genomes that are outliers, in terms of their sequencing or sample characteristics. Researchers can also use these techniques to identify the genomic sex of participants and compare them with their clinical notes. AI has improved the ability to process genomes rapidly and to high standards and can also now help improve genome interpretation.
Machine-learning-based AI requires substantial amounts of data for training. To address this, Genomics England has developed a platform to capture information and actions from automated genome interpretation systems and healthcare professionals. This data is carefully stored, to make it readily available for learning. It now hold observations on over 6 million variants across more than 35,000 cases. Going forwards, Genomics England anticipate developing models which can classify variants according to their likelihood of being pathogenic. However, this is likely to require more data, given the complexity of variant-disease interactions.
The research community has also started using AI based tools within the Genomics England research environment (which currently contains over 100,000 genomes and over 2.5 billion clinical data points). For example, researchers are using machine learning methods to identify genetic mutation signatures associated with certain cancers, helping to identify and classify sub-types of the disease and to identify targets for new treatments. This has the potential to improve cancer diagnosis by making better predictions of disease progression, and to better stratify patients for more effective and efficient treatment. This could be key to delivering on the promises of the long-term plan to diagnose three-quarters of all cancers at stage 1 or 2 by 2028 (27). Similar work is ongoing with rare disease, to identify driver mutations of particular conditions. Developing models will be able to better predict the severity of outcomes based on genomic and clinical data.
Whilst AI can undoubtedly increase the scale and speed of data collection and analysis, and accelerate progress, we should be careful to supervise machine learning. People still have a unique ability to critically evaluate and moderate learnings, which is vital to maintaining integrity and direction, and to avoid misinterpretation of data in making care decisions.
Genomics England, recognises the very privileged position it holds to have access to such enormous amounts of data, and to have the opportunity to work with new techniques such as AI to take genomic medicine to the next level. With this privilege comes huge responsibility. As outlined in the introduction patient data and related research demand the highest levels of privacy and protection, and ensuring this is a priority for the whole AI ecosystem. Similarly, safety is crucial and it is vital that the system is able to guarantee the integrity in the diagnoses and treatment plans which are delivered to patients, whether these are facilitated by AI, or more traditional methods. In order to build an optimal and sustainable future for genomic medicine, earning, building and retaining trust with patients, the public and technology and research partners, will be essential.
Despite tremendous progress in recent years, there is significant opportunity for AI and machine learning tools to make further advances in genomics, presenting an unparalleled opportunity to transform the application of genomic medicine in healthcare across the world.
EMRAD
( Simon Harris & Dierdre Dineen )
The East Midlands Radiology Consortium (EMRAD) is a partnership of seven NHS trusts spread over 11 hospitals, covering more than five million patients. EMRAD launched in 2013 with the objective to create a new, common digital radiology system. Its work was supported with the award of ‘Vanguard’ status by NHS England’s New Care Models programme which ran from 2016 to 2018.
This pioneering work saw the East Midlands become the first health community in the UK where NHS hospitals could quickly and easily share diagnostic images such as x-rays and scans. The cloud-based image-sharing system has set the national benchmark for a new model of clinical collaboration within radiology services in the NHS.
In 2018, EMRAD formed a partnership with two UK-based Artificial Intelligence (AI) companies, Faculty and Kheiron Medical Technologies , to help develop, test and - ultimately - deploy AI tools in the breast cancer screening programme in the East Midlands. The project is one of seven ‘wave two’ NHS Test Beds, and is administered by NHS England and the Office for Life Sciences. The Test Bed project is focused on Capacity, Care, and Confidence: it aims to improve and optimise clinical service capacity, to enhance patient care at significant scale and to increase NHS confidence in the utilisation of innovative machine learning tools. The project aims to develop and test both clinical and non-clinical (operational) AI tools. Kheiron’s ‘Mia(™)’ tool has the potential to support the clinical workforce issues in the service by acting as the second reader in the dual-read mammography workflow, while Faculty’s ‘Platform’ software has the potential to help optimise operational processes such as clinic scheduling and staff resourcing.
Clinical applications of AI
At the June EMRAD AI Project Board, the Lincolnshire BSP lead described the pressures as “tremendous”, with “no end in sight”. Deep learning is increasingly being proposed as a solution to the breast cancer screening workforce crisis. As part of their role in the Test Bed, Kheiron are conducting a large-scale retrospective study on mammograms from two of the NHS sites within the EMRAD Consortium. The aim is to test the generalisability of Mia™, their novel deep learning mammography software. They hope to conclude that their generalisable model is suitable for consideration as an independent reader in double-read screening programmes. This would have significant implications for the future of the breast screening workforce throughout the UK.
Non-clinical (operational) applications of AI
As well as using AI to address a clinical task, the Test Bed project is seeking to apply AI tools and techniques to the operational and administrative aspects of the breast screening programme, considering how AI can help to run the service in the most efficient and effective way possible. Faculty’s role in the Test Bed is to test the potential application in the breast screening programme of process optimisation tools and techniques developed on their ‘Platform’ software and pioneered in complex operational environments such as airlines, railways and high-street retailers. The aim is to make the best possible use of scarce resources like radiologists’ time and expensive machinery, and to reduce stress on the clinical and administrative workforce delivering the programme.
The Test Bed is working within the current service definition: it aims to deliver exactly the same breast screening programme in a more efficient way. For example, we will be training and testing an AI-powered intelligent capacity and demand planner. This should help screening round managers to more easily and accurately identify likely pinch-points where demand exceeds the service’s capacity, to identify ways to mitigate these pressures, and to simulate and estimate the likely knock-on impact of – for example – unplanned machinery or site down-time, or workforce changes, on the maintenance of the round length. We will also be testing a dashboard which aims to help programme managers identify their most and least efficiently used clinics, helping to best target constrained staff resources.
Discovery work for the Test Bed also identified opportunities to use AI tools to accurately predict which screening clients would and would not attend their screening appointment, potentially allowing for more accurate over-booking of clinics without detriment to the service levels individual screening clients receive. Similar techniques could be used to identify the screening appointment slot which an individual client is most likely to accept - boosting first-time acceptance rates, reducing DNAs, and reducing the administrative workload which is currently created by clients phoning to re-book their appointment for a more convenient time. Techniques developed in the marketing sector could even be used to test and optimise the communications sent to screening clients to maximise uptake and attendance rates.
Beyond the core screening clinics, AI tools may also be able to provide an early warning of increases in demand for assessment clinics, helping service managers to proactively schedule extra clinics before a spike in demand. Or these tools could predict the range of diagnostic tests which an attendee at a symptomatic clinic is likely to need, helping to schedule slots within a clinic to reduce clients’ waiting times.
If the structure and specification of the breast screening programme were to evolve in the future, this could open up further opportunities to make use of AI tools. For example, service capacity across a region is currently split into silos based on the allocation of GP practices to specific screening sites. If, in future, a ‘next test due date’ approach were adopted, this could potentially allow clients to be called for screening at a location that optimised their travel time and the available service capacity.
Realising all these opportunities will depend significantly on the availability and accessibility of the data which is required to train AI tools. Finding ways to securely and safely share appropriately de-identified client-level data for the purposes of service improvement will be vital. Building functional, modern connectivity into the core breast screening programme software will also be essential: programme managers already have to contend with a variety of IT systems, so core service delivery systems will need to be able to connect seamlessly with new AI tools if those tools are to be deployed effectively in the real world.
Cogstack
(James Teo)
King’s College Hospital NHS Foundation Trust, together with the South London and Maudsley Hospital, have developed an opensource real-time data warehousing tool called ‘Cogstack’ that improves operational efficiency significantly.
Cogstack meets the acute need for a more efficient way to clinically code to improve financial and operational efficiencies for providers throughout NHS.
Reading health text for clinical coding underpins operational, financial and forward planning activity throughout the NHS, but there is a significant source of data quality issues in the NHS (Capita, 2014). This is because Clinical Coding as a domain is under significant pressure due to the expanding volume of data in the past decade, resulting in incomplete data capture, and manpower shortages of skilled clinical coders. Big Data techniques may be a solution – search technology, web analytical techniques like natural language processing (NLP) and semantic artificial intelligence (AI) tools that can read and interpret electronic free text at scale.
These advances in NLP are accelerating, and Microsoft, Google and Amazon have now all expressed publicly that they are exploring the NLP and search technology use-cases in healthcare. This has the potential to accelerate the efficiency of clinical coders in terms of depth of coding as well as release staff for more complex tasks.
The team at King’s College Hospital NHS Foundation Trust and the South London and Maudsley Hospital tested Cogstack for clinical coding in a fracture outpatient clinic setting to identify undercoding and was able to triple the depth of coding within a month (from ~10% of cases to 30% of cases having procedures recorded accurately). This translates to £1,260,000 of financial activity per annum even without the efficiency gains. Using similar methods to Google, Apple Siri and Amazon Alexa, the team have developed further advanced prototype NLP algorithms for performing large-scale tagging of clinical text (Semantic EHR; MedCAT) with machine learning; this has the potential to code all clinical data in real-time with associated efficiency gains.
Cogstack is already functioning and deployed in a large NHS Trust, and has been tested with a real-world NHS problem showing it has potential for substantial disruption of manual healthcare processes. Further accelerated development is being proposed to expand the use of such NHS-grown open-source technologies in the NHS. Near-term benefits would be to improve efficiency of business intelligence and operational tasks initially, and allow release of human resource for more complex tasks. Subsequent medium-term and long-term benefits would emerge as the AI systems develop an understanding of medical language, revolutionising how clinical staff interact with computers.
Lessons from Estonia and Finland
(Tina Woods and Melissa Ream)
The AHSN Network AI programme took a group of senior stakeholders on a Study Tour to Estonia and Finland in April 2019 to take learnings back to the UK on creating a citizen-led open health & care data ecosystem.
Why these two countries? Estonia is considered the most advanced digital society in the world and has built an efficient, secure and transparent ecosystem that saves time and money (estimated to be 2% of GDP per year). Estonia’s e-citizen programme provides innovative e-solutions via a secure citizen ID and has demonstrated that an open and transparent attitude provides a good foundation for trust between the citizen and the state, and gives more control to the real owner of the data, the citizen. Finland is harnessing the power of data to benefit its citizens, having recently passed legislation on secondary use of data. It endorses ethical use of data, through its work on IHAN (International Human Account Network)- comparable to the IBAN standard used in banking but serving the ‘human driven data economy’ instead- and development of European guidelines for ethical use of data. SITRA, the Finnish innovation agency, is working with Estonia on two data exchange layer pilots to test the Estonia’s X-Road solution to provide social and healthcare services The key learnings from this Study Tour to take back into the UK scenarios are as follows: • Engaging citizens and building their trust in government has been central to the success of digital innovation in Finland and Estonia. • The development of a data infrastructure to support effective data exchange, democratise access to date and leverage integrated datasets has been fundamental to realising the potential of data-driven technologies. • An open approach to collaboration has been a key driver for change.
NHS-R Community
R is an open source tool for data-science and statistical analysis, employed by many different organisations including BBC News, Heathrow Ltd, GSK, The FT and BT for their data science needs.
Now, the NHS-R Community, established in 2017, from a standing start with support from The Health Foundation’s Advancing Applied Analytics Award, is dedicated to promoting the learning, application and use of R in the NHS. By providing problemoriented workshops across the country and a dedicated website with hundreds of subscribers the NHS-R Community promotes a positive attitude towards peer reviewing and learning. Helping to share efficiencies for members who have cut time spent on common tasks from weeks to hours and stimulate a wider conversation about what analysts can contribute to health care. Currently the NHS-R Community is working on producing an open library of validated, off-the-shelf solutions for others to adapt and use in healthcare.
NHSX Mental Health
NHSX has partnered with NHS England to work on meaningful challenges within the mental health space. The first is to look into children and young people’s mental health, specifically around preparation for future appointments, waiting times and nonattendance rates do not attend/was not brought rates. The team includes subject matter experts alongside NHSX colleagues who bring user-centered design practices and are dedicated to not only working on a significant problem but also raising capability of colleagues through exposing new ways of working. The team is researching with users, working in the open and proactive in sharing what they have found. They will discover pain points in the children and young people’s mental health services, outline future opportunities and constraints and recommend what to do next.
Optimam
Intelligent diagnostic support has advanced significantly in the areas of image recognition. Not only is image recognition a relatively mature area of artificial intelligence, but the structured digital datasets that are now common in radiology has facilitated the rapid training of algorithms to detect a number of pathologies. Despite this, there is a need for large AI research databases to ensure that models trained are robust and do not overfit their training data, or reproduce bias present in small datasets.
For this reason, over the last 10 years the Royal County Surrey Hospital has developed the knowledge, processes and bespoke tools to create large-scale medical image research databases, primarily aimed for use in AI and in the area of breast screening, nuclear medicine, MRI, tomosynthesis and radiogenomics.
The flagship database, OPTIMAM, focuses on 2D Full-Field Digital Mammography (FFDM) screening images, focussing on over-capacity breast screening programmes nationally and internationally. With 2.5 million de-identified images with associated clinical data and cancer location information remote PACS software).
This cloud-based database is currently shared with over 50 groups and companies working on AI. With complex sharing tools to manage the sharing of huge volumes of data, including comprehensive tracking and audit logs it is easy to manage data governance and ensure that training and test sets, do not overlap.
Survey case studies
Advancing Applied Analytics
Established in 2017, the Advancing Applied Analytics awards have awarded approximately £2.5m to 33 projects across the UK that seek to apply innovative approaches to building capability in the health and care system over 15 months. An additional £1.5m has been committed by the Health Foundation to fund up to 24 more projects over the coming years.
The programme funds projects that seek to address the capability deficiencies identified in the Foundation’s Understanding analytical capability in health and care report. The projects thus far have taken heterogeneous approaches, ranging from apprenticeships and training programmes to develop models and tools for clinicians and managers to better plan and deliver care.
An evaluation of the awards found that each project saw improvements in analytical capability that would not have been seen without the seed funding including the use of novel tools (deep learning, systems thinking), development of specific analytics techniques, learning new software skills (R, systems modelling), creation of new development opportunities, formation of new networks and collaborations that enabled analytics.
These awards have demonstrated that small seed funding is an effective way to promote innovation and catalyse analytical capability in the NHS, ultimately improving health care and wellbeing across the UK.
axial3D
Each person is unique, and each operation will be too, more-so in the cases of complex or novel procedures. A complex surgical procedure involving variant anatomy may be the first of its type seen by a surgeon, even in a specialist centre, and providing surgeons with the ability to anticipate and manage for the operation in advance can have a great impact on outcomes.
axial3D is helping to transform surgery by providing previously unavailable insights to clinicians for preoperative planning across the world. axial3D produces highly accurate 3D printed models of specific parts of the patient’s own anatomy. Based around patient scans, axial3D uses machine learning-based segmentation algorithms to automatically produce patient-specific 3D anatomical models which can be created and delivered in 24-48 hours. These anatomical models can be used by surgeons to plan for complex procedures, gain patient consent and minimise inter-clinician variability.
This results in better clinical outcomes for patients, enhanced insights for surgeons, and reduced time and costs for the surgery. Patients are also much better informed about their condition ahead of the proposed surgery when a 3D printed model is used in gaining consent.
BrainPatch
Transcranial electrotherapy uses small pulsed currents of electricity, delivered in a non-invasive manner, to influence cortical brain activity. It is hoped that through modulating cortical activity and promoting certain patterns, this technique can be used to influence learning, mood and other brain functions.
BrainPatch is a neurotechnology start-up working on a solution based on low current non-invasive brain stimulation, already shown to have positive effects in mental health, depression and diseases such as epilepsy and Alzheimer’s. By using AI to optimise the stimulation protocols to each individual it may be possible to achieve better efficacy with no additional risk for a variety of medical and non-medical everyday applications. Brain Patch intends to use smart contracts, allowing developers and consumers to prioritise their own goals, making use of their hard-and soft-ware to fulfil them.
Chief AI
Whilst AI poses to transform healthcare, the digital receptiveness of existing healthcare bodies stands to challenge its rapid and effective adoption. Current structures of access and procurement are not designed for digital processes, further slowing down the speed of adaptation and innovation in this area.
Chief AI is commissioned to create an intelligent marketplace where third party AI algorithms can be provisioned on demand, leading to efficiency and convergence in the uptake of AI within the healthcare ecosystem. Moreover, Chief AI’s own products provide novel diagnostics and drug discovery services powered by artificial intelligence and machine learning. Trained on world class medical datasets from around the world, Chief AI creates efficiency in the global management of non-communicable diseases such as cancer by developing and deploying medical diagnostic models, informing and customising oncology treatments, and identifying novel biomarkers for precision medicine.
Concentric Health
Ensuring that patients understand the reasons and consequences for decisions made about their health forms the backbone, of an auditable and accountable healthcare system, as well as being a necessary pre-requisite to empowering people to make better decisions about their health.
Welsh health-tech start up, Concentric Health’s, vision is to ensure all decisions are informed by patient outcomes and shared by patient and clinician. Their fully auditable digital consent platform uses data to personalise outcomes, is designed to simplify complexity and ensures that patients are informed and engaged throughout their journey.
eTrauma
In busy, fast paced, clinical environments it can be difficult to keep on top of the administrative task of ensuring every patient is met with the highest standard of care. Around the whiteboards, paper-notes and ward lists care has to be delivered in a standard, accountable way, ensuring that no one is missed and the service works efficiently, ensuring that the max number of people can be seen. No-where is this more true than in the emergency and acute services.
eTrauma is a bespoke cloud-based patient management system that has been used to manage over 500,000 patients in 15 acute trusts. The platform is hosted within the N3 / HSCN network and is used as an end-to-end clinical management system for Trauma and Orthopaedic departments.
Our use of cloud-based, learning natural language processing algorithms, and structured granular data sets has helped to significantly improve data quality in patients with acute traumatic orthopaedic injuries. Leveraging this detailed data, we have partnered with NHS trusts to develop new models of care such as a revolutionary digital fracture liaison service, which automatically detects patients who may benefit from secondary osteoporosis prevention at the time of initial referral, thus improving both efficiency and capture of patients that may benefit from preventative treatment strategies.
In addition, by facilitating service redesign and improved efficiency, our systems have helped NHS trusts reduce referral to assessment times for orthopaedic fracture patients from 2 weeks to 2 days, injury to theatre times by up to 7 days, and saving over 1,000 clinic appointments in a single NHS trust alone.
First Derm
Skin diseases are amongst the most common diseases encountered by health professionals, with 13 million skin related GP consultations per year. Despite this, they are relatively overlooked in medical education, leaving many GPs unequipped to deal with the quantity and diversity of presentations they may see. These ailments have the potential to be life-threatening, or even psychologically harmful, given their highly visible nature, and the goal of providing high quality care to all has become harder in the context of an ageing population with dwindling resources and staffing shortages.
First Derm aims to Transition from a human powered dermatology service to a faster and more accurate artificial intelligence (AI) algorithm driven service empowering patients to access high quality care through their phones.
Over the last 5 years First Derm has collected a unique dataset of over 350k+ “amateur” smartphone dermatology images and we are adding another 15 000 images per month. The AI API in dermatology (https:// www.firstderm.com/ai-dermatology/) can screen 43 common skin diseases, from STDs to skin cancer. The algorithms are now 50% accurate on one disease and 80% correct including top 3 skin diseases (differential answers). The AI is a good fit for an anonymous quick screening of a skin concern that will let you know what next steps to take and as a decision support tool for virtual care clinics.
The service is in 7 different languages and has users from over 160 and is currently being integrated into online STD testing websites, messaging apps such as Microsoft Teams and WeChat, and online health services like abi.ai
Forms4Health
Many healthcare providers operate with paper notes, records, and forms of data collection. This format is not only expensive, environmentally damaging and incompatible with rapid iterative design at a systems level, but also requires time and work to manage, store and analyse.
Forms4Health is an intuitive, easy to integrate, electronic smart forms platform, facilitating paperless working across health and social care. We believe clinical software needs to reflect the variety and dynamic nature of the healthcare sector. The design of forms4health is responsive, versatile and interoperable to the complex digital needs of the Health and Social Care profession, allowing an organisation to quickly and easily design their own smart forms. These may be used to create forms for any purpose, supporting workflows across all departments can be used by staff to produce complex resources with very little training including a range of sophisticated features, from branching logic (conditional questions), calculations, to clinical decision support.
In addition to removing the need for paper forms, reducing costs, and saving time, the data gathered through Forms4Health can be easily analysed to provide valuable business intelligence, from evaluating patient wait times, to assessing resource allocation. Benefits realised for our customers include improving care outcomes through accurate up to date information recorded at the point of care, supporting patient engagement, enhancing information sharing across internal and external departments and care settings, connecting existing systems to deliver care, improving data quality through standardisation, ease of completion and consistent inputs and saving clinical and patient time driving efficiency.
Google Health
A turning point has been reached in AI for healthcare: not only is more and better research emerging all the time, we are closer than ever before to applying this research to real-world clinical practice and to proving out benefits for patients and clinicians. That’s why we’ve formed Google Health: to bring cutting-edge AI research into clinical practice. Google Health brings together many teams from across the organisation, including the team that was formerly DeepMind Health.
One example is our work with Moorfields Eye Hospital. The DeepMind Health team began working with Moorfields in 2016 to apply machine learning approaches to diagnosing common eye disease from routine scans. Initial research from the partnership showed that the AI system could triage urgent OCT scans as accurately as leading retina specialists. Crucially, it didn’t miss a single urgent case. This research has now been transferred over to Google Health, as of September 2019, and we are now working with Moorfields to validate this research on a wider population and ultimately to deploy the system into clinical practice.
Streams, our mobile medical assistant app for clinicians, is another interesting example. It was developed by the DeepMind Health team in collaboration with clinicians at the Royal Free London NHS Foundation Trust to help identify patients at risk of acute kidney injury (AKI). The app uses the existing national AKI algorithm to flag patient deterioration and supports the review of clinical information at the bedside.
An independent evaluation showed that the app speeds up detection and treatment and prevents missed cases. Thanks to the app, clinicians were able to respond to urgent AKI cases in 14 minutes or less - a process which, using existing systems, might otherwise have taken many hours. The Trust, as data controller, remains in control over personal data at all times and the team can only process it in line with their instructions. Although it does not yet use AI, Streams demonstrates the potential to surface predictive insights at the moment when clinicians need them--with life-saving consequences. The app, and the team working on it, also transitioned over to Google Health in September 2019.
iRhythm Technologies
Detecting cardiac dysrhythmias can be difficult in practice; with a wide variety of problems, ranging from innocuous ectopic and skipped beats, to life threatening arrhythmias. To differentiate in practice, a record of the event is needed, which can be hard to gather, often requiring expensive and repeated testing, often without fruition.
iRhythm Technologies is a leading digital health care company redefining the way cardiac arrhythmias are clinically diagnosed. Our Zio service provides uninterrupted, comprehensive monitoring to accurately and efficiently detect and diagnose heart conditions, such as arrhythmias, at the first time of asking. The company combines wearable biosensor devices worn for up to 14 days and cloud-based data analytics with powerful proprietary algorithms that distil data from millions of heartbeats into clinically actionable information. Published studies have shown improvements in arrhythmia detection and characterisation have the potential to transform clinical management of patients – eliminating the requirement for numerous patient visits due to indeterminate repeat tests and significantly improving the patient experience.
Kaido
The Physical and Mental Health of employees is an increasingly important strategic priority for the modern employer due to its link with productivity and engagement.
In the NHS, this has a direct correlation with the quality and standard of care that is delivered to patients.
Through a combination of fun and engaging company wide challenges, tailored health and wellbeing content and bespoke management reporting, Kaido empowers employees to take control of their wellbeing, whilst supporting NHS employers in building a happier, healthier and more positive place to work. Kaido is already having a profound impact across the NHS working with employers such as The Royal Marsden Hospital NHS Foundation Trust, Great Ormond Street Hospital NHS Foundation Trust and North West Ambulance service and to date NHS employees have completed a massive 30,000,000 minutes of physical activity on the platform, and report a decrease in work-related stress (37%), an improved mood (40%) and increased workplace motivation (51%).
Kortical
Blood products have a short shelf life; in fact, blood platelets only last only 7 days. Ensuring all hospitals have a supply of the different blood types, at all times, is a complex problem, which involves understanding supply, manufacturing, distribution, stock holding, logistics and hospital demand levels, and necessitates an amount of waste.
Kortical is using their platform to build Artificial Intelligence (AI)/Machine Learning (ML) models to predict demand and supply levels, allowing for patient needs to be met more precisely, reducing ad-hoc transport costs and improving efficiencies across the board.
Kortical have built an AI-powered platform to predict supply and demand for platelets for all the hospitals in England, which also takes into account diverse data such as weather, bank holidays, and understands that different trusts require different types of blood products, given the large regional differences.
The AI predicts supply, which varies with who comes in, and which blood type and volume of platelets they donate, as well as demand, 1 to 7 days out, and finally the platform optimises for logistics. All this to ensure that there are the right levels in the right place and demand is met with a little contingency on top to keep the nation covered. Testing against historical data the AI platform is performing better than previous demand and supply forecasting methods by over 10% and in the first quarter of 2020 Kortical will be able to release the figures in production, demonstrating their ability to reduce cost and waste without compromising patient care.
Lifelight
Vital signs monitoring forms the basis of a clinician’s view of a patient, informing them over time about their baseline health and trajectory of change. Previously this information has been available through regular observations in a healthcare context, taking valuable staff time; however, patients have not been empowered to gather their own observations in a clinically valid way, and as such in the community this perspective is largely unavailable.
Lifelight is a unique software technology that measures blood pressure, heart rate, respiration and oxygen saturation in just 40 seconds simply by a patient looking into the camera on a standard smartphone or tablet. No cuff, wearables or contact needed. Lifelight’s computer-vision, machine learning, algorithms are trained on data from an 8,500 patient clinical study at Portsmouth Hospitals Trust which recorded over 1 million heartbeats.
Validated to clinical grade, Lifelight allows rapid triage in ED and urgent care, fast, contactless ward observations and ultimately, the ability for any patient at home to measure their own vital signs simply with their smartphone as part of 111 calls, remote consultations and outpatient appointments.
My Cognition
Poor cognitive fitness is a major component and a risk factor in mental illness. Long-term conditions have a negative impact on patients’ mental health, their everyday activities, social relationships and self-management. 30-40% of people with a chronic condition have a mental health problem and conversely 46% of people with a mental health problem have a longterm physical health problem. Either way 50% - 90% of both populations will experience clinically defined cognitive impairment, which is impacting their healthcare outcomes and how well they manage their condition, this becomes more disabling as we age.
MyCognition App strengthens patient cognitive health, protects against cognitive decline, develops mental resilience and prevents and treats mental illness. Occupationally it promotes independence, improves quality of life and ability to self-manage physical health. The App measures, monitors and tracks cognitive health with its clinically validated 15-min digital cognitive assessment MyCQ, and then corrects cognitive deficits with a personalised training video game, AquaSnap. Healthy habits are encouraged with its positive behavioural and lifestyle change programme.
Roche Diabetes Care Platform
Nearly 1 in 10 people worldwide have diabetes, and the proportion is rising. This chronic condition covers a large number of causes; however, most common is Type 2 diabetes, closely linked to diet, weight and lifestyle. Despite its high prevalence, this condition has a high impact on morbidity, being a major cause of blindness, kidney failure, heart attacks, stroke and lower limb amputation worldwide. The management of diabetes is complex and requires high levels of clinical coordination as well as patient understanding and engagement to ensure that short and long term complications do not ensue.
Roche Diabetes Care Platform is an online modular diabetes management platform that quickly connects you to actionable insights for a more personalized approach to diabetes care. The streamlined workflow of the platform allows for fast insights into key health and diabetes data, contributing to a clear and holistic picture of patient health. Moreover the platform aims to engage patients and promote better understanding and positive behaviour change.
Sensyne Health
The wealth of data generated in the healthcare system today poses many opportunities for improved care and increased efficiencies.
Sensyne health creates value from accelerating the discovery and development of new medicines and improving patient care through the analysis of real-world evidence from large databases of anonymised patient data in collaboration with NHS Trusts. Currently with four validated products in employment, Sensyne health works closely with clinical partners to ensure that products designed are fit for purpose in a clinical environment, making use of and working towards well defined clinically validated metrics. GDm-Health and EDGE empower patients to manage gestational diabetes and COPD more effectively in the community, whilst also facilitating the collection and analysis of relevant data.
Together these applications save money and clinician time through helping patients avoid urgent and emergency hospital admission by helping to improve their understanding and management of their conditions, as well as providing simple, easy and effective digital contact to their clinicians.
SEND makes use of routine vital signs monitoring to calculate a dynamic risk score for patients, predicting likelihood of deterioration and allowing clinicians to monitor and manage a population of patients effectively, allocating resources based on clinical need. Moreover the analytic capacity of their products is employed in research and has to date identified a distinct phenotype of patients experiencing blood-pressure rises in the early morning, placing them at higher risk of an adverse event. .
Sentinel
“On average patients are monitored only once every 8 hours on general wards and significantly less when discharged home. The UK NHS discharges 16.3m patients p.a. of which 14% will be readmitted as an emergency admission within 30 days of discharge. This has a significant increased cost impact and results in increased mortality. These figures are broadly similar across the E.U.”
As a consortium of university hospitals concluded, ”Patients die because signs of deterioration are missed. There is a huge unfulfilled need for better monitoring of vital signs to identify high-risk patients who are on general hospital wards or at home.”
The Universities calculated that they will save €4.3m for every 30k patients discharged if high-risk patients are continuously monitored in hospitals and at home, post-discharge. Sentinel provides a continuous wearable monitor, currently undergoing MHRA conformity assessments, for known patterns in the patients’ vital-signs every 60 seconds, 24x7. In doing so, Sentinel can detect if new conditions are developing or if the patient is deteriorating before the situation develops into an emergency, thus allowing for faster and more effective treatment.
Storm ID
The management of chronic diseases occupies an ever larger proportion of hospital productivity. These complex and often co-morbid conditions provide an opportunity to develop new models of care allowing for intelligent predictive and preventative management in the community, ensuring that hospital resources are used to their maximum efficiency. In the case of Chronic Obstructive Pulmonary Disorder (COPD), the second most common cause of emergency hospital admissions in the UK, accounting for 1 in 8 of all hospital admissions, the need is well defined.
This chronic respiratory condition affects 1.2 million people in the UK and is forecast by the WHO to become the third leading cause of death worldwide by 2030. With the cost of emergency admission and management highthis new service combines patient generated health data, with clinical details pulled dynamically from electronic health records to present a real time view of COPD patients to care teams. Using predictive analytics, the service aims to stratify those patients at highest risk of exacerbation to prioritise those for intervention and prevent an emergency hospital admission. The service offers patients access to their personalised self management plan, details of standard and emergency medication and two way secure messaging with care team.
Storm ID have also launched new outpatient digital appointments services across NHS Trusts in Scotland which moves routine outpatient care to an asynchronous, virtual model that can be delivered up to 40% more efficiently. We are collaborating with NHS on new digital care pathways with integrated machine learning for Stroke, Sleep Apnoea and have a Retinopathy service in early planning stages. We believe that virtual care models will transform the routine treatment of chronic conditions to a management by exception care model where machine learning and rules based models will identify those patients in need of intervention.
Veye Chest
Lung cancer is the most common cause of cancer in men, and the third most common cause of cancer in the UK. Given that three quarters of lung cancers in the UK are detected at a late stage, lung cancer is often fatal and contributes to a large national and global burden of mortality.
Aidence has developed Veye Chest to aid early diagnosis of lung cancer on chest-CT scans. Their software automatically detects and evaluates pulmonary nodules, compares the patient’s current scan to prior imaging, and produces an automated report for the radiologist. Their machine learning algorithms were trained using 45,000 chest-CT scans (NLST dataset) and a subsequent clinical validation study of VeyeChest was performed by the University of Edinburgh.
Since receiving CE-certification, VeyeChest has been running in clinical practice in more than 10 sites across the UK and wider Europe. Aidence’s goals are to help improve efficiency in radiology departments, accuracy in early lung cancer detection, and to contribute to the NHS achieving successful and sustainable targeted lung health check programmes.
About NHSX and this report
NHSX brings teams from the Department of Health and Social Care, NHS England and NHS Improvement together into one unit to drive digital transformation and lead policy, implementation and change. NHSX is responsible for delivering the Health Secretary’s Tech Vision, building on the NHS Long Term Plan by focusing on five missions:
- Reducing the burden on clinicians and staff, so they can focus on patients
- Giving people the tools to access information and services directly
- Ensuring clinical information can be safely accessed, wherever it is needed
- Improving patient safety across the NHS
- Improving NHS productivity with digital technology
Although this report has named editors, it results from the collective effort of a great number of individuals who kindly gave up their time to contribute their thoughts, ideas and research. A full list of acknowledgements is provided at the end of the report. There are, however, several key organisations, and individuals who provided input without which this report would not have been possible. With this in mind, we would like to thank: Tina Woods, Collider Health; Melissa Ream, Marie-Anne Demestihas and Sile Hertz, AHSN Network; Anna Steere, NHSX; Dr. Sam Roberts, Accelerated Access Collaborative.
References
Acknowledgements
We would like to thank each individual person who contributed to the writing, editing, designing and publishing of this report.